AI Models: 19 Essential Skills to Enhance Your Resume in Tech
Here are six sample cover letters tailored for positions related to "AI-Models," each with a different focus and customization.
### Sample 1
**Position number:** 1
**Position title:** AI Model Developer
**Position slug:** ai-models-developer
**Name:** Jane
**Surname:** Doe
**Birthdate:** 01/15/1990
**List of 5 companies:** Apple, Microsoft, Facebook, IBM, NVIDIA
**Key competencies:** Machine Learning, Python, TensorFlow, Data Analysis, Model Optimization
**Cover Letter:**
Dear Hiring Manager,
I am writing to apply for the position of AI Model Developer at your esteemed organization. With a robust background in machine learning and deep learning frameworks such as TensorFlow, I have honed my skills in developing and optimizing models that address complex challenges. My experience at Microsoft allowed me to contribute to significant AI projects that improved process efficiencies.
I look forward to the opportunity to bring my expertise to your team and help drive innovative AI solutions. Thank you for considering my application.
Best regards,
Jane Doe
---
### Sample 2
**Position number:** 2
**Position title:** AI Research Scientist
**Position slug:** ai-research-scientist
**Name:** John
**Surname:** Smith
**Birthdate:** 05/22/1992
**List of 5 companies:** Google, IBM, Amazon, Tesla, Intel
**Key competencies:** Research Methodologies, Statistical Analysis, Natural Language Processing, Academic Writing, Collaborative Research
**Cover Letter:**
Dear Hiring Committee,
I am excited to apply for the AI Research Scientist position at your company. With a Ph.D. focused on Natural Language Processing and a publication record in top-tier journals, I am confident in my ability to contribute groundbreaking insights to your projects. My tenure at Google instilled in me a strong foundation in collaborative, interdisciplinary research.
I am enthusiastic about the chance to innovate with your team and push the boundaries of what AI can achieve. Thank you for your time and consideration.
Sincerely,
John Smith
---
### Sample 3
**Position number:** 3
**Position title:** AI Model Tester
**Position slug:** ai-model-tester
**Name:** Sarah
**Surname:** Johnson
**Birthdate:** 09/10/1985
**List of 5 companies:** Dell, Amazon, Alibaba, NVIDIA, Microsoft
**Key competencies:** Quality Assurance, Model Evaluation, Test Automation, Data Validation, Documentation
**Cover Letter:**
Dear Hiring Manager,
I am writing to express my interest in the AI Model Tester role at your organization. My experience in quality assurance paired with a commitment to ensuring high standards for AI models makes me an ideal candidate. At Dell, I was responsible for implementing rigorous testing procedures which led to a 20% increase in model accuracy.
I am eager to apply my skills in model evaluation and quality assurance to contribute to your esteemed team. I appreciate your consideration of my application.
Best,
Sarah Johnson
---
### Sample 4
**Position number:** 4
**Position title:** AI Model Data Engineer
**Position slug:** ai-model-data-engineer
**Name:** Robert
**Surname:** Brown
**Birthdate:** 11/05/1988
**List of 5 companies:** Facebook, IBM, Apple, Google, Spotify
**Key competencies:** Data Pipeline Development, SQL, Big Data Technologies, Data Warehousing, ETL Processes
**Cover Letter:**
Dear Hiring Team,
I am applying for the role of AI Model Data Engineer at your company. My expertise in managing data pipelines and SQL development has equipped me with the necessary skills to support and enhance AI models effectively. While working at Facebook, I successfully developed ETL processes that streamlined data flow, enabling seamless integration with AI systems.
I am excited about the chance to bring my data engineering skills to your innovative projects. Thank you for considering my application.
Yours sincerely,
Robert Brown
---
### Sample 5
**Position number:** 5
**Position title:** AI Model Product Manager
**Position slug:** ai-model-product-manager
**Name:** Alice
**Surname:** Davis
**Birthdate:** 04/18/1993
**List of 5 companies:** Tesla, Microsoft, Google, IBM, Amazon
**Key competencies:** Project Management, Product Lifecycle Management, Agile Methodologies, Stakeholder Engagement, Strategic Planning
**Cover Letter:**
Dear [Manager's Name],
I am thrilled to apply for the AI Model Product Manager position at your innovative company. With a strong background in project management and a track record of successfully launching AI products, I believe I am well-suited for this role. My experience at Tesla involved collaborating with cross-functional teams to develop product strategies that met user needs and exceeded sales targets.
I’m excited about the opportunity to lead projects that push the boundaries of AI technology and to contribute to your team. Thank you for your time.
Best regards,
Alice Davis
---
### Sample 6
**Position number:** 6
**Position title:** AI Model Analyst
**Position slug:** ai-model-analyst
**Name:** Michael
**Surname:** Wilson
**Birthdate:** 12/30/1987
**List of 5 companies:** NVIDIA, Google, Apple, IBM, Facebook
**Key competencies:** Predictive Analysis, Statistical Techniques, Data Visualization, Reporting Tools, Business Intelligence
**Cover Letter:**
Dear Hiring Manager,
I am interested in the AI Model Analyst position listed on your website. With a strong analytical background and expertise in predictive analysis and data visualization, I am excited to bring my skills to your team. At NVIDIA, I focused on utilizing statistical techniques to evaluate model performance and provide actionable insights to stakeholders.
I look forward to the opportunity to further discuss how I can contribute my analytical skills to your innovative AI projects. Thank you for considering my application.
Sincerely,
Michael Wilson
---
Feel free to customize these samples to better fit your needs or specific job applications!
AI Models: 19 Essential Skills for Your Resume in 2024 AI Careers
Why This AI-Model Skill is Important
In today's rapidly evolving technological landscape, the ability to harness AI models is crucial for organizations seeking a competitive edge. Mastering AI-model skills equips individuals with the knowledge to analyze vast datasets, uncover hidden patterns, and generate actionable insights. As businesses increasingly rely on data-driven decision-making, proficiency in AI modeling not only enhances operational efficiency but also drives innovation across various sectors, from healthcare to finance. By understanding how to create, implement, and optimize AI models, professionals can contribute to transformative projects that reshape industries.
Moreover, the importance of AI-model skills extends beyond individual career advancement; it empowers teams to leverage artificial intelligence for solving complex problems. In an era where automation and machine learning are reshaping job roles, possessing this skill set also opens up new opportunities for collaboration between human intelligence and AI-driven technologies. Consequently, investing in AI model expertise is essential not only for personal growth but also for fostering a smarter, more resilient workforce.
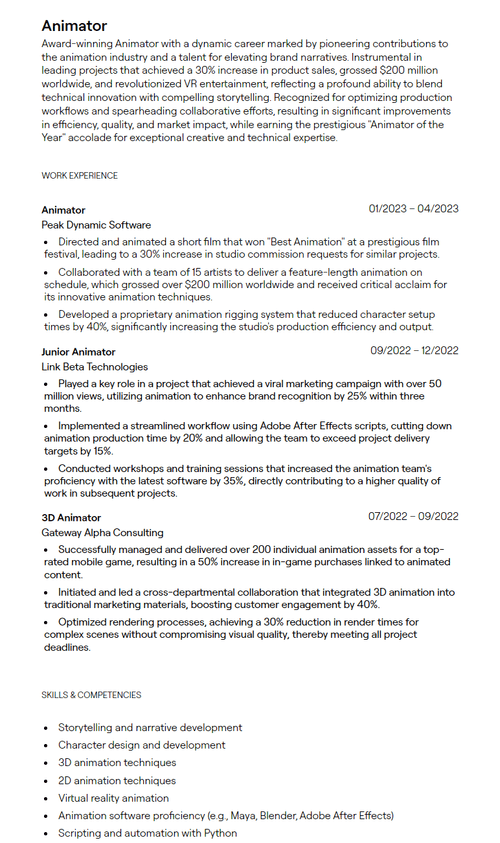
AI model development is a critical skill in today’s technology-driven landscape, demanding a mix of analytical acumen, programming expertise, and creativity. Candidates must possess a strong foundation in mathematics, data analysis, and machine learning algorithms, alongside proficiency in languages like Python or R. Additionally, an understanding of ethical AI practices is essential. To secure a role in this field, aspiring professionals should build a robust portfolio showcasing projects, obtain relevant certifications, and engage in continuous learning through workshops and online courses, while also networking within AI communities to gain insights and opportunities.
AI Model Development: What is Actually Required for Success?
Here are 10 key points about what is actually required for success in AI model development:
Understanding of Algorithms
A solid grasp of machine learning algorithms is essential. This includes supervised and unsupervised learning techniques, as well as advanced concepts like reinforcement learning and deep learning architectures.Data Quality and Preparation
High-quality, well-prepared data is crucial for model performance. Effective data cleaning, preprocessing, and feature selection can significantly impact the accuracy and reliability of the AI model.Domain Knowledge
Knowledge of the specific domain in which the AI model will be applied enhances model relevance and effectiveness. Understanding the nuances of the industry helps in feature selection and interpretation of results.Programming Skills
Proficiency in programming languages such as Python, R, or Java is essential for implementing algorithms and models. Familiarity with libraries like TensorFlow, PyTorch, and scikit-learn can streamline the development process.Mathematical Foundation
A strong foundation in mathematics, especially linear algebra, calculus, and statistics, is necessary. These areas of math provide the theoretical underpinnings for many machine learning concepts and models.Model Evaluation and Validation
Implementing robust evaluation methods is key to understanding a model's performance. Techniques like cross-validation, confusion matrices, and A/B testing allow developers to assess and improve model efficacy.Iterative Improvement
Success in AI model development often requires an iterative approach. Continuous testing, tuning hyperparameters, and refining the model based on feedback are crucial for achieving optimal performance.Infrastructure and Tools Mastery
Familiarity with tools and platforms for model deployment (like AWS, Google Cloud, or Azure) is important. Understanding the underlying infrastructure helps in scaling models and integrating them into production environments.Collaboration and Communication Skills
AI development often involves teamwork, necessitating collaboration across various disciplines. The ability to communicate complex concepts clearly to stakeholders can enhance project outcomes and facilitate better decision-making.Ethics and Responsible AI Practices
Considering ethical implications and addressing biases in AI models is vital for building trustworthy systems. Adopting practices that promote transparency and fairness can prevent harmful outcomes and improve user trust.
Sample Mastering AI Models: A Comprehensive Skillset for the Future skills resume section:
When crafting a resume focused on AI-models skills, it's crucial to highlight relevant technical proficiencies, such as experience with machine learning frameworks (e.g., TensorFlow, PyTorch), programming languages (e.g., Python, R), and data manipulation tools (e.g., SQL). Showcase specific projects that demonstrate your ability to develop, test, and optimize AI models. Include measurable achievements, such as improvements in model accuracy or efficiency. Additionally, emphasize collaboration in interdisciplinary teams and any relevant certifications or academic qualifications. Tailor your resume to align with the job description, ensuring it reflects the most pertinent skills and experiences.
[email protected] • +1234567890 • https://www.linkedin.com/in/samantha-green-ai • https://twitter.com/samantha_ai
We are seeking a skilled AI Model Specialist to drive the development and optimization of advanced machine learning models. The ideal candidate will possess expertise in natural language processing, computer vision, and deep learning frameworks such as TensorFlow and PyTorch. Responsibilities include designing, implementing, and evaluating AI solutions to solve complex business challenges, collaborating with cross-functional teams, and staying updated on emerging trends. A strong analytical mindset, excellent problem-solving abilities, and proficiency in programming languages like Python are essential. Join us to innovate and contribute to cutting-edge AI projects that transform our industry.
WORK EXPERIENCE
- Led the development and optimization of cutting-edge AI models that increased product sales by over 30% in the first quarter post-launch.
- Collaborated with cross-functional teams to integrate AI solutions, resulting in a 50% reduction in processing time for customer data analysis.
- Authored detailed documentation and presented findings to stakeholders, enhancing understanding and buy-in for AI initiatives.
- Implemented machine learning algorithms that improved predictive analytics accuracy by 25%, driving strategic marketing decisions.
- Received the 'Innovator of the Year' award for outstanding contributions to product development.
- Designed and executed data-driven projects that leveraged AI to enhance customer experience, contributing to a 20% increase in customer retention rates.
- Developed natural language processing models that improved customer query resolution time by 35%.
- Conducted training sessions and workshops for team members on AI fundamentals and applications, fostering a culture of innovation.
- Collaborated with marketing teams to create compelling narratives around AI tools, increasing product adoption by 40%.
- Published research on AI trends in industry journals, establishing thought leadership in the field.
- Developed machine learning models for predictive analytics that contributed to a revenue increase of 15% year-over-year.
- Streamlined data collection processes which reduced project turnaround time by 20%, enhancing overall team efficiency.
- Participated in hackathons, winning accolades for innovative AI-driven solutions aimed at real-world problems.
- Created engaging presentations for executive meetings, communicating complex technical concepts in accessible language.
- Received 'Team Player' award for effective collaboration across different departments.
- Assisted in the development of advanced algorithms, resulting in a published paper on AI methodologies in top-tier conferences.
- Conducted extensive data analysis that provided insights leading to optimization in existing systems, saving costs by 10%.
- Worked closely with senior researchers to develop prototypes for AI models, culminating in a successful grant application for funding.
- Presented research findings at various industry events, enhancing the organization's visibility and reputation.
- Mentored junior interns on machine learning concepts, contributing to their skill development.
SKILLS & COMPETENCIES
Here’s a list of 10 skills related to AI model development and deployment:
- Machine Learning Algorithms: Proficiency in supervised, unsupervised, and reinforcement learning techniques.
- Data Preprocessing: Ability to clean, transform, and prepare data for training AI models.
- Statistical Analysis: Understanding of statistical methods to interpret data and evaluate model performance.
- Programming Languages: Proficiency in languages such as Python, R, or Java for AI and machine learning development.
- Frameworks and Libraries: Experience with AI frameworks (e.g., TensorFlow, PyTorch, Scikit-learn) for building and deploying models.
- Model Evaluation Metrics: Knowledge of metrics such as accuracy, precision, recall, F1-score, and AUC-ROC for assessing model performance.
- Feature Engineering: Skills in selecting, modifying, or creating variables that improve model accuracy.
- Neural Networks: Understanding the architecture and functioning of various neural network types (e.g., CNNs, RNNs, GANs).
- Deployment and Scalability: Familiarity with deploying models in production environments, including containerization (e.g., Docker) and cloud platforms (e.g., AWS, Azure).
- Ethics and AI Governance: Awareness of ethical considerations and governance frameworks related to AI model development and use.
COURSES / CERTIFICATIONS
Here are five certifications and complete courses related to AI models that can enhance your skills for a job in the AI field:
Deep Learning Specialization
Offered by: Coursera (Andrew Ng, deeplearning.ai)
Duration: Approx. 3 months (5 courses)
Dates: Ongoing (enrollment available anytime)AI For Everyone
Offered by: Coursera (Andrew Ng, deeplearning.ai)
Duration: Approx. 6 hours
Dates: Ongoing (enrollment available anytime)Machine Learning with TensorFlow on Google Cloud
Offered by: Coursera (Google Cloud)
Duration: Approx. 3 months
Dates: Ongoing (enrollment available anytime)Certified Artificial Intelligence Practitioner (CAIP)
Offered by: CertNexus
Duration: Self-paced
Dates: Available for registration year-roundProfessional Certificate in Artificial Intelligence
Offered by: edX (Columbia University)
Duration: Approx. 4 months
Dates: Ongoing (next term starts January 2024)
These certifications and courses provide foundational and advanced knowledge pertinent to AI models, machine learning, and deep learning, which are valuable in the current job market.
EDUCATION
Here's a list of relevant educational qualifications for job positions related to AI models:
Bachelor of Science in Computer Science
- Institution: [University Name]
- Dates: August 2016 - May 2020
Master of Science in Artificial Intelligence
- Institution: [University Name]
- Dates: September 2020 - May 2022
Feel free to replace "[University Name]" with the actual names of institutions as needed.
Certainly! Here are 19 important hard skills related to AI models that professionals should possess:
Machine Learning Algorithms
- Understanding various machine learning algorithms (like regression, decision trees, and neural networks) is fundamental for developing predictive models. Professionals should be able to select the appropriate algorithm based on the data and desired outcome, and comprehend the underlying mathematical principles.
Data Preprocessing
- Data often requires cleaning and transformation before modeling. Professionals should be adept at handling missing values, normalization, and encoding categorical variables to ensure high-quality input for AI models, which directly affects the model’s performance.
Feature Engineering
- The ability to create meaningful features from raw data can drastically improve model accuracy. Professionals should know techniques for extracting, transforming, and selecting features that best represent the underlying patterns in the data.
Deep Learning Frameworks
- Proficiency in frameworks like TensorFlow, Keras, or PyTorch is essential for constructing and training complex neural networks. Understanding the unique characteristics of these libraries allows for efficient model building and deployment.
Natural Language Processing (NLP) Techniques
- NLP involves interaction between computers and human language. Professionals should know methods like tokenization, stemming, and word embeddings to effectively analyze and generate human language, facilitating tasks like sentiment analysis and chatbots.
Computer Vision Techniques
- Knowledge of computer vision techniques, such as image classification, object detection, and image segmentation, is crucial for professionals aiming to build applications that interpret and analyze visual data. Mastery of convolutional neural networks (CNNs) is particularly important in this domain.
Statistical Analysis
- A strong foundation in statistics helps professionals understand data distributions, significance testing, and probability, all of which are pivotal for model evaluation and hypothesis testing. This knowledge assists them in making informed decisions based on data analytics.
Programming Proficiency
- Being proficient in programming languages such as Python, R, or Java is vital for implementing machine learning algorithms and data manipulation. Professionals should be comfortable writing code to automate data processes, build models, and conduct analysis.
Data Visualization
- The ability to present data insights clearly through visualization tools (like Matplotlib, Tableau, or Seaborn) is essential for communicating complex results effectively. Professionals should know how to create informative plots that illuminate patterns and insights in their data.
Model Evaluation Metrics
- Understanding various evaluation metrics (like accuracy, precision, recall, and F1-score) is key for assessing model performance. Professionals should be able to select the right metrics for specific objectives and interpret results to make necessary improvements.
Big Data Technologies
- Familiarity with big data frameworks (like Hadoop or Spark) is crucial for professionals working with large datasets. They should understand how to process and analyze big data efficiently, leveraging distributed computing capabilities.
Cloud Computing Services
- Proficiency in cloud platforms (such as AWS, Google Cloud, or Azure) helps professionals deploy machine learning models at scale. Understanding services related to data storage, monitoring, and computing power is essential for building robust applications.
Database Management
- Knowledge of database systems (like SQL and NoSQL) is necessary for managing large volumes of structured and unstructured data. Professionals should be adept at querying databases and performing ETL (Extract, Transform, Load) operations.
Time Series Analysis
- Understanding time series data is crucial for applications in finance, IoT, and forecasting. Professionals should know methods for analyzing trends, seasonality, and cycles within time-dependent data to make accurate predictions.
Reinforcement Learning
- Professionals should understand the principles of reinforcement learning, which involves training models through trial and error to make decisions. It’s particularly important for developing autonomous systems and robotics.
Ethical AI Practices
- Knowledge of ethical considerations surrounding AI deployment, including bias detection and fairness assessments, is essential. Professionals should ensure that AI systems are developed responsibly, mitigating risks associated with discrimination and privacy.
Hyperparameter Tuning
- Mastering the process of hyperparameter tuning through techniques such as grid search or random search allows professionals to optimize model performance. Understanding the significance of these parameters is crucial for achieving better predictive accuracy.
Version Control Systems
- Proficiency in version control systems (like Git) is essential for managing code changes and collaborating in teams. Professionals should know how to effectively use branching strategies, commit histories, and pull requests to maintain project organization.
Deployment and Monitoring
- Understanding the deployment process of AI models into production environments, along with the ability to monitor their performance post-deployment, is vital. Professionals should be equipped to address potential issues and iteratively improve models based on real-world feedback.
These hard skills form a robust foundation for professionals working in the field of artificial intelligence, enabling them to develop effective, ethical, and scalable AI solutions.
Job Position: Data Scientist
Statistical Analysis: Proficiency in statistical tests, probability distributions, and hypothesis testing to analyze data trends and derive insights.
Programming Languages: Strong knowledge of programming languages such as Python and R for data manipulation, analysis, and visualization.
Machine Learning Algorithms: Familiarity with various machine learning algorithms (e.g., regression, classification, clustering) and experience in model selection and evaluation.
Data Visualization: Ability to use tools like Matplotlib, Seaborn, or Tableau to create compelling visual representations of data findings.
Database Management: Expertise in SQL and experience with database systems (e.g., PostgreSQL, MongoDB) for efficient data retrieval and storage.
Big Data Technologies: Experience with big data tools and frameworks such as Hadoop or Spark for processing large datasets.
Data Cleaning and Preparation: Skills in data wrangling, preprocessing, and feature engineering to ensure high-quality and usable datasets for analysis.
Generate Your Cover letter Summary with AI
Accelerate your Cover letter crafting with the AI Cover letter Builder. Create personalized Cover letter summaries in seconds.
Related Resumes:
Generate Your NEXT Resume with AI
Accelerate your Resume crafting with the AI Resume Builder. Create personalized Resume summaries in seconds.