Data Pipelines: 19 Essential Skills to Enhance Your Resume for Analytics
Certainly! Here are six sample cover letters for subpositions related to the "data-pipelines" role. Each sample includes the required fields.
---
**Sample 1**
**Position number:** 1
**Position title:** Data Pipeline Engineer
**Position slug:** data-pipeline-engineer
**Name:** John
**Surname:** Doe
**Birthdate:** 1990-05-14
**List of 5 companies:** Apple, Dell, Google, Amazon, Microsoft
**Key competencies:** ETL processes, SQL proficiency, Python programming, Apache Airflow, Data warehousing
**Cover Letter:**
[Your Address]
[City, State, Zip]
[Email Address]
[Phone Number]
[Date]
Hiring Manager
[Company Name]
[Company Address]
[City, State, Zip]
Dear Hiring Manager,
I am writing to express my interest in the Data Pipeline Engineer position at [Company Name], as advertised. With extensive experience in ETL processes and a strong command of SQL, I am excited about the opportunity to contribute to your team.
As a seasoned professional with expertise in Python programming and Apache Airflow, I have successfully developed and maintained data pipelines that improve the efficiency and reliability of data processing. At my previous role at [Previous Company], I streamlined data integration processes which resulted in a 30% increase in system performance.
I am particularly drawn to [Company Name] due to your commitment to data-driven decision-making. I would love the opportunity to leverage my skills in data warehousing to enhance your operations.
Thank you for considering my application. I look forward to the possibility of discussing my application further.
Sincerely,
John Doe
---
**Sample 2**
**Position number:** 2
**Position title:** Data Engineer
**Position slug:** data-engineer
**Name:** Sarah
**Surname:** Smith
**Birthdate:** 1992-09-23
**List of 5 companies:** Google, Facebook, Twitter, IBM, Salesforce
**Key competencies:** NoSQL databases, Hadoop ecosystem, Data modeling, Cloud computing, Spark
**Cover Letter:**
[Your Address]
[City, State, Zip]
[Email Address]
[Phone Number]
[Date]
Hiring Manager
[Company Name]
[Company Address]
[City, State, Zip]
Dear Hiring Manager,
I am excited to apply for the Data Engineer position at [Company Name]. My background in the Hadoop ecosystem and NoSQL databases equips me with a unique skill set to build efficient data pipelines that can handle large volumes of data.
At [Previous Company], I successfully implemented optimized data models and migrated systems to cloud computing platforms, which improved our data retrieval times by 40%. I am highly proficient in using Apache Spark for large-scale data processing, a skill I believe would be beneficial to your team.
I admire [Company Name]'s innovative approach to data solutions and would be thrilled to contribute to your mission. Thank you for considering my application; I look forward to discussing this exciting opportunity with you.
Best regards,
Sarah Smith
---
**Sample 3**
**Position number:** 3
**Position title:** Data Pipeline Architect
**Position slug:** data-pipeline-architect
**Name:** Michael
**Surname:** Johnson
**Birthdate:** 1988-01-30
**List of 5 companies:** Amazon, Netflix, LinkedIn, Oracle, SAP
**Key competencies:** System architecture design, Data integration, Scalability, Performance tuning, Data governance
**Cover Letter:**
[Your Address]
[City, State, Zip]
[Email Address]
[Phone Number]
[Date]
Hiring Manager
[Company Name]
[Company Address]
[City, State, Zip]
Dear Hiring Manager,
I am writing to apply for the Data Pipeline Architect position at [Company Name]. With a proven track record in system architecture design and data integration, I am confident in my ability to create high-performing data pipelines tailored to your needs.
In my previous work at [Previous Company], I led a team that improved our data governance protocols, resulting in a 50% reduction in data processing errors. My focus on scalability and performance tuning ensures that data systems not only meet current demands but are also prepared for future growth.
I am inspired by [Company Name]’s dedication to innovation and would be eager to bring my expertise to your esteemed team. Thank you for your consideration; I look forward to the opportunity for further discussion.
Sincerely,
Michael Johnson
---
**Sample 4**
**Position number:** 4
**Position title:** ETL Developer
**Position slug:** etl-developer
**Name:** Jessica
**Surname:** Brown
**Birthdate:** 1995-07-19
**List of 5 companies:** Google, Dell, HP, Cisco, Yahoo
**Key competencies:** ETL tools, Data profiling, Data quality management, Data migration, Business intelligence
**Cover Letter:**
[Your Address]
[City, State, Zip]
[Email Address]
[Phone Number]
[Date]
Hiring Manager
[Company Name]
[Company Address]
[City, State, Zip]
Dear Hiring Manager,
I am excited to submit my application for the ETL Developer position at [Company Name]. My experience with various ETL tools and data quality management makes me a great fit for this role.
At [Previous Company], I was responsible for designing data workflows and conducting thorough data profiling to ensure integrity and accuracy. My efforts resulted in improved data migration strategies that significantly reduced downtime.
I am particularly impressed by [Company Name]'s commitment to leveraging data for business intelligence and would be eager to contribute my skills to such meaningful projects. Thank you for considering my application; I hope to discuss this opportunity with you soon.
Warm regards,
Jessica Brown
---
**Sample 5**
**Position number:** 5
**Position title:** Data Analytics Pipeline Specialist
**Position slug:** data-analytics-pipeline-specialist
**Name:** David
**Surname:** Wilson
**Birthdate:** 1993-11-05
**List of 5 companies:** Airbnb, Spotify, Snapchat, Tumblr, Reddit
**Key competencies:** Analytical tools, Visualization software, Data extraction, Statistical analysis, Collaboration
**Cover Letter:**
[Your Address]
[City, State, Zip]
[Email Address]
[Phone Number]
[Date]
Hiring Manager
[Company Name]
[Company Address]
[City, State, Zip]
Dear Hiring Manager,
I am writing to apply for the Data Analytics Pipeline Specialist position at [Company Name]. With a robust background in analytical tools and visualization software, I am skilled in transforming raw data into insightful information.
At [Previous Company], I developed a comprehensive data extraction process that enhanced our analytical capabilities, resulting in actionable insights for business decisions. My collaborative approach ensures that cross-departmental needs are considered during data processing.
I admire [Company Name]'s innovative use of analytics and would love to be part of a team making such a substantial impact. Thank you for your time and consideration; I look forward to the possibility of further discussions.
Best,
David Wilson
---
**Sample 6**
**Position number:** 6
**Position title:** Big Data Engineer
**Position slug:** big-data-engineer
**Name:** Emily
**Surname:** Garcia
**Birthdate:** 1994-04-09
**List of 5 companies:** Facebook, IBM, Salesforce, Accenture, Square
**Key competencies:** Big Data technologies, Data lake implementation, Data mining, Machine learning, Distributed systems
**Cover Letter:**
[Your Address]
[City, State, Zip]
[Email Address]
[Phone Number]
[Date]
Hiring Manager
[Company Name]
[Company Address]
[City, State, Zip]
Dear Hiring Manager,
I am thrilled to apply for the Big Data Engineer position at [Company Name]. My expertise in Big Data technologies and data lake implementation positions me to contribute effectively to your projects.
While working at [Previous Company], I implemented a data mining strategy that optimized our data retrieval processes and improved our machine learning models’ accuracy. My experience with distributed systems ensures that I can manage complex data architectures with ease.
I find [Company Name]’s vision of data utilization inspiring and would be honored to be part of such an innovative team. Thank you for considering my application; I look forward to the opportunity to discuss how I can contribute.
Sincerely,
Emily Garcia
---
Feel free to customize any of these letters to better fit your profile or the specific job you are applying for!
Data Pipelines: 19 Essential Skills to Boost Your Resume in 2024
Why This Data-Pipelines Skill is Important
In today's data-driven landscape, mastering data-pipelines is crucial for any organization aiming to leverage its data assets effectively. Data pipelines facilitate the seamless flow of data from various sources to storage and analysis systems, enabling businesses to make informed decisions based on real-time insights. With the volume, variety, and velocity of data increasing, the ability to efficiently process and transform data is vital for operational efficiency and strategic planning.
Additionally, proficiency in data-pipelines enhances an individual's technical skill set, making them invaluable in roles such as data engineering, data science, and analytics. This skill encompasses knowledge of ETL (Extract, Transform, Load) processes, data integration, and the use of various tools and technologies like Apache Kafka, Apache Airflow, and cloud services. By mastering data pipelines, professionals can ensure data quality, reduce latency, and enable better collaboration across teams, ultimately driving business growth and innovation.
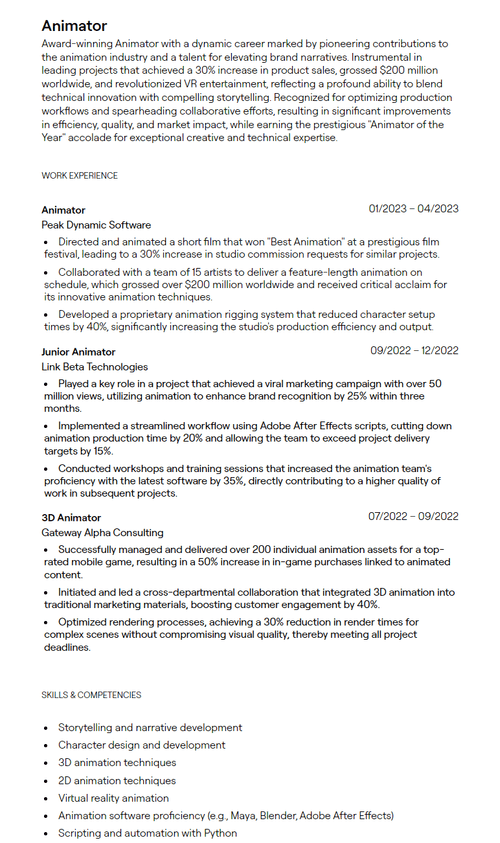
Data pipeline skills are essential for efficiently moving, processing, and analyzing data in today's data-driven landscape. Professionals in this role must possess strong programming abilities, proficiency with data integration tools, and a solid understanding of database management and ETL (Extract, Transform, Load) processes. Critical thinking and problem-solving skills are necessary to optimize workflows and ensure data integrity. To secure a job in this field, candidates should build a robust portfolio showcasing relevant projects, gain experience with key technologies such as Apache Kafka or Airflow, and pursue certifications in data engineering to enhance their qualifications and appeal to prospective employers.
Data Pipeline Development: What is Actually Required for Success?
Here are ten key points detailing what is actually required for success in developing data pipelines:
Understanding Data Sources
A solid grasp of various data sources, such as relational databases, APIs, and streaming services, is crucial. Knowing how to connect, extract, and manipulate data from these sources forms the foundation of any data pipeline.Data Transformation Skills
Proficiency in data transformation techniques is essential, as raw data often needs to be cleaned and structured. This includes understanding concepts like ETL (Extract, Transform, Load) and familiarity with transformation tools and frameworks.Knowledge of Pipeline Orchestration
Understanding how to manage and schedule tasks within a data pipeline is key to ensuring efficient processing. Utilizing orchestration tools like Apache Airflow or Luigi can help automate and monitor workflows effectively.Proficiency in Programming Languages
Familiarity with programming languages such as Python, SQL, or Scala is vital for writing scripts to extract, process, and load data. The ability to manipulate data programmatically enhances the flexibility and functionality of your pipelines.Data Warehousing Concepts
Understanding data warehousing principles helps in determining how structured data should be stored and accessed. Knowledge of platforms like Google BigQuery, Amazon Redshift, or Snowflake aids in designing robust data storage solutions.Version Control Familiarity
Utilizing version control systems like Git is important for collaboration and tracking changes in code. This practice ensures that pipeline development is organized and that multiple team members can contribute without conflicts.Performance Tuning and Optimization
Knowledge of techniques for optimizing data pipeline performance is critical to handle large datasets efficiently. This includes optimizing queries, ensuring parallel processing, and reducing redundancy to improve data loading times.Monitoring and Logging Practices
Implementing monitoring and logging within the data pipeline allows for proactive issue detection. Tools like ELK Stack or Prometheus can help track the health and performance of the pipeline, enabling swift response to failures.Data Security and Compliance
Understanding data governance and compliance frameworks is crucial for protecting sensitive information. Ensuring that pipelines adhere to regulatory standards (e.g., GDPR or HIPAA) safeguards data integrity and security.Soft Skills and Collaboration
Strong communication and collaboration skills are necessary for working effectively within cross-functional teams. The ability to convey technical concepts to non-technical stakeholders enhances team synergy and project success.
Each of these points contributes to building a comprehensive skill set essential for success in creating and maintaining data pipelines.
Sample Mastering Data Pipelines: From Ingestion to Insight skills resume section:
null
[email protected] • +1-555-123-4567 • https://www.linkedin.com/in/alicejohnson/ • https://twitter.com/alice_johnson
We are seeking a skilled Data Pipeline Engineer to design, implement, and optimize robust data pipelines for our organization. The ideal candidate will have expertise in ETL processes, data integration, and cloud technologies. You will collaborate with cross-functional teams to gather requirements, ensuring data is easily accessible and actionable. Proficiency in programming languages such as Python or Java, along with experience in data processing frameworks like Apache Spark or Airflow, is essential. Strong problem-solving abilities and a passion for data engineering will drive your success in enhancing data workflows and supporting data-driven decision-making across the company.
WORK EXPERIENCE
- Designed and implemented data pipelines that increased data processing efficiency by 40%.
- Led a cross-functional team to develop a data reporting platform that resulted in a 25% increase in actionable insights for stakeholders.
- Optimized ETL processes, reducing data latency by 30%, which enhanced real-time analytics capabilities.
- Conducted workshops on data storytelling, helping teams leverage data to drive strategic decision-making.
- Awarded 'Data Innovator of the Year' for pioneering projects that significantly boosted product sales.
- Developed and maintained data pipelines for integration across multiple platforms, contributing to a 20% increase in data accessibility.
- Collaborated with marketing teams to analyze user behavior, leading to the launch of a new product line that generated an additional $2M in revenue.
- Introduced a data visualization tool that helped non-technical stakeholders grasp complex data concepts easily.
- Spearheaded a project that automated report generation, reducing workload by 50% and improving report accuracy.
- Presented findings at the Annual Data Conference, enhancing the company’s reputation in the industry.
- Engineered data pipelines that supported a data warehouse migration, resulting in enhanced data integrity and accessibility.
- Mentored junior data team members, fostering skills in data processing and visualization.
- Implemented data quality checks that improved overall data reliability by 35%.
- Worked closely with product teams to align data strategy with business goals, which increased product sales by 15%.
- Published a technical paper on data pipeline efficiency in a leading industry journal.
- Created interactive dashboards that provided critical insights to the sales team, increasing conversion rates by 18%.
- Conducted thorough data analysis to identify trends, resulting in the optimization of marketing strategies.
- Enhanced reporting tools that decreased data retrieval times by 70%, empowering teams with real-time insights.
- Presented analytical findings to executive leadership, influencing key business decisions.
- Awarded 'Employee of the Year' for outstanding contributions to business intelligence projects.
SKILLS & COMPETENCIES
Here’s a list of 10 skills that are typically associated with a job position related to data pipelines:
ETL (Extract, Transform, Load) Proficiency: Experience with building and optimizing ETL processes to move data from various sources to storage solutions.
Data Modeling: Ability to design and implement data models that efficiently structure and organize data for processing and analysis.
SQL Proficiency: Strong skills in SQL for querying databases, performing data manipulation, and ensuring data integrity.
Big Data Technologies: Familiarity with big data frameworks such as Hadoop, Spark, or Flink for handling large-scale data processing.
Cloud Data Services: Understanding of cloud platforms (e.g., AWS, Azure, GCP) and their data services for building scalable data pipelines.
Data Warehousing Solutions: Knowledge of data warehousing technologies (e.g., Redshift, Snowflake, BigQuery) for integrating and storing large volumes of data.
Scripting and Programming Languages: Proficiency in programming languages such as Python, Java, or Scala for data manipulation and pipeline automation.
Data Governance and Quality Assurance: Skills in implementing data quality checks and governance practices to ensure data reliability and compliance.
Workflow Orchestration Tools: Experience with tools like Apache Airflow, Luigi, or Apache NiFi for managing and scheduling data workflows.
Monitoring and Debugging: Ability to monitor data pipelines, identify issues, and implement solutions to ensure high availability and performance.
COURSES / CERTIFICATIONS
Certainly! Here’s a list of five certifications and complete courses that are related to data pipeline skills:
Google Cloud Professional Data Engineer Certification
Date: Ongoing enrollment, with next exam dates available quarterly
Provides knowledge on designing, building, and operationalizing data processing systems on Google Cloud.Microsoft Azure Data Engineer Associate (DP-203) Certification
Date: Ongoing, with exams available throughout the year
Focuses on integrating, transforming, and consolidating data from various sources into structures that are suitable for analysis.Data Engineering Nanodegree by Udacity
Date: Self-paced, typically completed in 3-6 months
Covers the design and building of data pipelines using tools like Apache Airflow and Spark.IBM Data Engineering Professional Certificate
Date: Self-paced, usually completed within 3-6 months
A comprehensive program covering the essential skills for data engineering, including data pipelines with Python and SQL.Coursera Data Warehousing for Business Intelligence Specialization
Date: Self-paced, typically completed in 4-5 months
Focuses on data warehousing, ETL processes, and tools to create data architectures for analysis.
Make sure to check the specific platforms for enrollment dates and availability!
EDUCATION
Here’s a list of relevant educational qualifications related to data pipeline skills:
Bachelor's Degree in Computer Science
- Institution: XYZ University
- Dates: September 2015 - June 2019
Master's Degree in Data Science
- Institution: ABC University
- Dates: September 2019 - June 2021
Feel free to ask if you need anything else!
Here are 19 important hard skills that professionals working with data pipelines should possess, along with brief descriptions for each:
Data Modeling
- Understanding how to create and manage data models is crucial for structuring data effectively. This involves designing schemas that optimize data storage and retrieval while aligning with business requirements and use cases.
ETL (Extract, Transform, Load)
- Proficiency in ETL processes is essential for moving data from various sources to a data warehouse. Professionals should be skilled in extracting raw data, transforming it to meet analysis needs, and loading it into target systems.
SQL (Structured Query Language)
- SQL is the backbone of data manipulation and retrieval in relational database systems. Proficiency in SQL allows professionals to query databases effectively, perform data analysis, and create complex reports.
Data Warehousing
- Knowledge of data warehousing concepts is important for integrating and managing large volumes of data. Professionals should understand data warehousing architectures, techniques for data storage, and how to ensure data integrity and availability.
Big Data Technologies
- Familiarity with big data frameworks like Hadoop, Spark, or Kafka is essential for processing large datasets efficiently. Professionals need to leverage these technologies for scalable data processing and real-time analytics.
Data Integration Tools
- Expertise in data integration platforms (e.g., Apache NiFi, Talend, Informatica) is necessary for connecting disparate data sources. Professionals should be able to design data flows that ensure seamless data movement and transformation.
Scripting Languages (Python, R, etc.)
- Proficiency in scripting languages such as Python and R enables automation of data pipeline tasks. These languages are instrumental in data manipulation, analysis, and building custom functions for specific data processing needs.
Cloud Computing
- Understanding cloud platforms (e.g., AWS, Azure, Google Cloud) helps professionals deploy scalable data pipelines. Knowledge of cloud storage and computing resources also aids in creating efficient environments for data processing.
Data Quality Assurance
- Ensuring data quality is critical for making accurate and reliable business decisions. Professionals should implement validation, cleansing, and profiling measures to maintain high standards of data integrity throughout the pipeline.
APIs (Application Programming Interfaces)
- Skills in working with APIs allow data professionals to pull or push data from various web services. Understanding RESTful and SOAP protocols is key to integrating external data sources into the pipeline smoothly.
Containerization (Docker, Kubernetes)
- Familiarity with containerization tools facilitates the deployment of applications and services in a consistent environment. This skill is vital for creating scalable and reproducible data pipeline environments.
Version Control (Git)
- Proficiency in version control systems like Git is essential for collaborating on code and managing changes. It enables data professionals to track modifications in data pipeline code and coordinate with team members effectively.
Data Security and Compliance
- Knowledge of data security protocols and compliance standards (e.g., GDPR, HIPAA) is crucial for protecting sensitive information. Professionals should be skilled in implementing security measures to safeguard data throughout its lifecycle.
Business Intelligence (BI) Tools
- Familiarity with BI tools (e.g., Tableau, Power BI) helps in visualizing and interpreting data pipelines’ output. Professionals should use these tools to generate actionable insights and reports to aid business decision-making.
Logging and Monitoring
- Understanding logging and monitoring techniques is essential for diagnosing issues in data pipelines. Professionals should implement robust monitoring systems to track pipeline performance and alert for any anomalies.
Data Lake Architecture
- Knowledge of data lake concepts allows professionals to manage unstructured data efficiently. Understanding how to integrate data lakes with existing data systems enhances flexibility in data storage and analysis.
Workflow Orchestration Tools
- Proficiency in tools like Apache Airflow or Luigi is essential for managing complex data workflows. These orchestration tools help automate and schedule tasks within the data pipeline for seamless operation.
Data Governance
- Understanding data governance frameworks ensures proper management of data assets. Professionals should be skilled in defining data ownership, data stewardship, and policies to maintain data quality and accessibility.
Machine Learning Integration
- Familiarity with machine learning concepts allows professionals to enrich data pipelines with predictive analytics. Understanding how to integrate ML models into pipelines enables organizations to leverage data for advanced decision-making.
These hard skills provide a strong foundation for professionals working in data pipelines to manage, process, and analyze data effectively to support business objectives.
Job Position Title: Data Engineer
SQL Proficiency: Strong ability to write complex SQL queries for data extraction, transformation, and analysis from relational databases.
ETL Processes: Expertise in designing and implementing Extract, Transform, Load (ETL) processes to move and transform data from various sources into a target data warehouse.
Data Pipeline Development: Experience in building and maintaining scalable data pipelines using tools like Apache Spark, Apache Airflow, or similar frameworks.
Big Data Technologies: Knowledge of big data technologies such as Hadoop, Hive, and Kafka for processing and managing large datasets.
Cloud Platforms: Familiarity with cloud data services (e.g., AWS, GCP, Azure) for data storage and processing, including tools like AWS S3, Redshift, or Google BigQuery.
Programming Languages: Proficient in programming languages commonly used for data manipulation and analysis, such as Python, Scala, or Java.
Data Modeling and Schema Design: Understanding of data modeling concepts and the ability to design effective schemas for both relational and non-relational databases.
Generate Your Cover letter Summary with AI
Accelerate your Cover letter crafting with the AI Cover letter Builder. Create personalized Cover letter summaries in seconds.
Related Resumes:
Generate Your NEXT Resume with AI
Accelerate your Resume crafting with the AI Resume Builder. Create personalized Resume summaries in seconds.