Deep Learning Skills for Your Resume: 19 Essential Expertise in AI
Certainly! Below are six different sample cover letters tailored for subpositions related to "deep learning." Each sample encapsulates a unique position while incorporating common key competencies in this field.
### Sample 1
**Position number**: 1
**Position title**: Deep Learning Engineer
**Position slug**: deep-learning-engineer
**Name**: John
**Surname**: Doe
**Birthdate**: 1990-01-15
**List of 5 companies**: Apple, Dell, Google, Microsoft, NVIDIA
**Key competencies**: Deep Learning Frameworks (TensorFlow, PyTorch), Python Programming, Neural Networks, Model Optimization, Data Preprocessing
---
**Cover Letter**:
[Your Address]
[City, State, Zip]
[Email Address]
[Phone Number]
[Date]
**Hiring Manager**
[Company Name]
[Company Address]
[City, State, Zip]
Dear Hiring Manager,
I am writing to express my interest in the Deep Learning Engineer position at [Company Name]. With a solid background in machine learning and a specialized focus on deep learning frameworks like TensorFlow and PyTorch, I am excited about the opportunity to contribute to your innovative projects.
Throughout my career, I have developed advanced neural network models and optimized them for performance using best practices in model architecture and data preprocessing. At my previous role with [Previous Company Name], I successfully improved our existing models, resulting in a 20% increase in prediction accuracy.
I am particularly drawn to [Company Name] because of your commitment to integrating AI solutions that enhance user experience. I look forward to discussing how my background in deep learning can contribute to your team's success.
Thank you for considering my application. I am looking forward to the opportunity to speak with you.
Sincerely,
John Doe
---
### Sample 2
**Position number**: 2
**Position title**: Machine Learning Scientist
**Position slug**: machine-learning-scientist
**Name**: Jane
**Surname**: Smith
**Birthdate**: 1992-03-22
**List of 5 companies**: Tesla, Google, Facebook, IBM, Amazon
**Key competencies**: Algorithm Development, Statistical Analysis, Python & R Programming, Data Visualization, Deep Learning
---
**Cover Letter**:
[Your Address]
[City, State, Zip]
[Email Address]
[Phone Number]
[Date]
**Hiring Manager**
[Company Name]
[Company Address]
[City, State, Zip]
Dear Hiring Manager,
I am excited to apply for the Machine Learning Scientist position at [Company Name]. With experience in developing and implementing cutting-edge algorithms, I believe my skills align well with the requirements of your team.
At [Previous Company Name], I focused on statistical analysis and data visualization to interpret complex data sets, which led to actionable insights for product development strategies. My expertise in deep learning technologies allows me to propose solutions that significantly impact data-driven decision-making.
I admire [Company Name] for its innovative approach to technology and believe my background can help push the boundaries of machine learning applications. I would welcome the opportunity to further discuss how my experience can benefit your organization.
Thank you for your time and consideration.
Best regards,
Jane Smith
---
### Sample 3
**Position number**: 3
**Position title**: Data Scientist - Deep Learning
**Position slug**: data-scientist-deep-learning
**Name**: Mike
**Surname**: Johnson
**Birthdate**: 1988-05-10
**List of 5 companies**: Nokia, Intel, Oracle, Twitter, Salesforce
**Key competencies**: Data Mining, Feature Engineering, Neural Network Architecture Design, SQL, Machine Learning Techniques
---
**Cover Letter**:
[Your Address]
[City, State, Zip]
[Email Address]
[Phone Number]
[Date]
**Hiring Manager**
[Company Name]
[Company Address]
[City, State, Zip]
Dear Hiring Manager,
I am writing to apply for the Data Scientist - Deep Learning position at [Company Name]. My seven years of experience in data mining and feature engineering make me an ideal candidate for your team.
In my last position at [Previous Company Name], I designed neural network architectures that improved our predictive modeling capabilities, resulting in a 30% enhancement in accuracy. My practical knowledge of machine learning techniques combined with proficiency in SQL allows me to effectively extract and analyze large data sets.
I am particularly impressed by [Company Name]’s commitment to utilizing deep learning for solutions that positively impact users. I would love the chance to explore how I can contribute to your ongoing projects.
Thank you for considering my application. I look forward to your response.
Sincerely,
Mike Johnson
---
### Sample 4
**Position number**: 4
**Position title**: AI Researcher - Deep Learning
**Position slug**: ai-researcher-deep-learning
**Name**: Emily
**Surname**: Davis
**Birthdate**: 1991-06-05
**List of 5 companies**: Microsoft, Tesla, Netflix, Adobe, Spotify
**Key competencies**: Research Methodologies, Literature Reviews, High-Performance Computing, Model Deployment, Advanced Statistical Analysis
---
**Cover Letter**:
[Your Address]
[City, State, Zip]
[Email Address]
[Phone Number]
[Date]
**Hiring Manager**
[Company Name]
[Company Address]
[City, State, Zip]
Dear Hiring Manager,
I am excited to submit my application for the AI Researcher - Deep Learning position at [Company Name]. With a strong foundation in research methodologies and high-performance computing, I am passionate about exploring innovative applications of deep learning.
At [Previous Company Name], I conducted extensive literature reviews and designed experiments that led to the development of novel deep learning models. My work not only advanced our research but also contributed to published papers in reputable journals.
I am particularly impressed with [Company Name]’s projects in AI and believe my background can help drive the innovation you strive for. I would love the opportunity to discuss how my research can align with your vision.
Thank you for your consideration.
Warm regards,
Emily Davis
---
### Sample 5
**Position number**: 5
**Position title**: Computer Vision Engineer
**Position slug**: computer-vision-engineer
**Name**: Alex
**Surname**: Martinez
**Birthdate**: 1989-10-01
**List of 5 companies**: NVIDIA, Amazon, Facebook, Uber, Siemens
**Key competencies**: Image Processing, CNNs, OpenCV, Python & C++, Algorithm Optimization, Data Annotation
---
**Cover Letter**:
[Your Address]
[City, State, Zip]
[Email Address]
[Phone Number]
[Date]
**Hiring Manager**
[Company Name]
[Company Address]
[City, State, Zip]
Dear Hiring Manager,
I am writing to express my interest in the Computer Vision Engineer position at [Company Name]. My extensive experience in image processing and convolutional neural networks (CNNs) would be an asset to your innovative team.
During my tenure at [Previous Company Name], I enhanced the performance of our computer vision algorithms, delivering a 25% decrease in processing time while improving accuracy. I am proficient in OpenCV and have a solid command of Python and C++, allowing me to contribute effectively to cross-functional projects.
I greatly admire [Company Name] for its commitment to cutting-edge technology and would be thrilled to be a part of your team working on transformative solutions. I look forward to the possibility of discussing my application further.
Thank you for your time.
Best,
Alex Martinez
---
### Sample 6
**Position number**: 6
**Position title**: Cloud AI Developer
**Position slug**: cloud-ai-developer
**Name**: Chloe
**Surname**: White
**Birthdate**: 1993-04-25
**List of 5 companies**: Google Cloud, Amazon Web Services, IBM Cloud, Oracle, Alibaba Cloud
**Key competencies**: Cloud Computing, Deep Learning APIs, Model Deployment, DevOps, Scalable Applications
---
**Cover Letter**:
[Your Address]
[City, State, Zip]
[Email Address]
[Phone Number]
[Date]
**Hiring Manager**
[Company Name]
[Company Address]
[City, State, Zip]
Dear Hiring Manager,
I am excited to apply for the Cloud AI Developer position at [Company Name]. With a strong background in cloud computing and deep learning, I am eager to bring my technical expertise to your cutting-edge team.
In my previous role at [Previous Company Name], I developed scalable applications utilizing cloud infrastructures that streamlined AI model deployment. My hands-on experience with various deep learning APIs allows me to effectively integrate AI services into cloud environments.
I am particularly impressed with [Company Name]’s dedication to leveraging AI solutions in cloud computing. I look forward to the chance to contribute to your pioneering projects and further discuss how I can be a valuable asset to your team.
Thank you for considering my application.
Sincerely,
Chloe White
---
These samples demonstrate a variety of specialties and skills related to deep learning, allowing for personalization as needed in real-world applications.
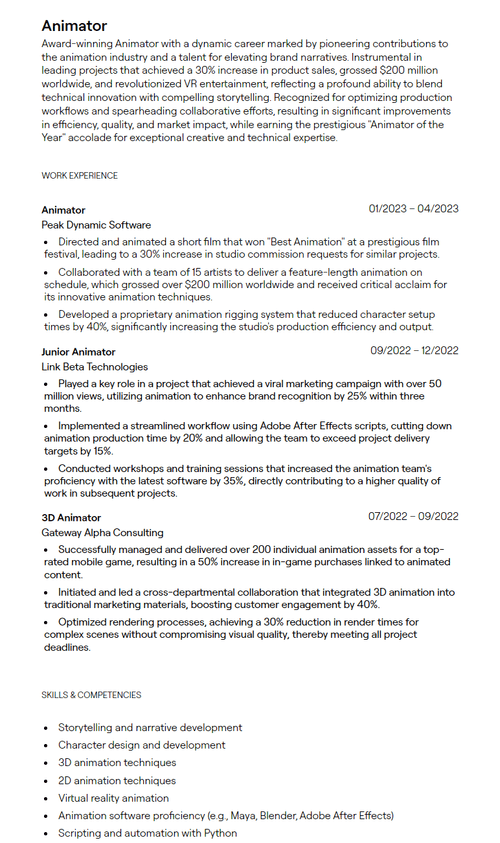
Sample skills resume section:
WORK EXPERIENCE
- Led a team to design and implement a deep learning model that improved product recommendation accuracy by 30%, resulting in a 20% increase in sales.
- Collaborated with cross-functional teams to integrate natural language processing algorithms into customer support systems, reducing response time by 40%.
- Developed and deployed a predictive analytics platform that generated actionable insights, contributing to a 15% boost in marketing ROI.
- Mentored junior data scientists and conducted training workshops on deep learning techniques, enhancing team skillsets and project outcomes.
- Presented key findings at industry conferences, garnering recognition and enhancing the company's visibility in the AI space.
- Worked on a machine learning project that optimized supply chain logistics, achieving a 25% reduction in operational costs.
- Utilized advanced deep learning frameworks to analyze customer behavior data, leading to personalized marketing campaigns that boosted engagement by 35%.
- Conducted A/B testing for algorithm adjustments, yielding insights that improved user experience and increased retention rates.
- Collaborated with software engineers to implement machine learning models into production, ensuring seamless integration and performance monitoring.
- Published findings and strategies in a peer-reviewed journal, establishing the company as a thought leader in the data science community.
- Pioneered research on generative adversarial networks (GANs), leading to the development of innovative applications in image synthesis.
- Implemented cutting-edge neural network architectures that enhanced model performance by 50% on benchmark datasets.
- Authored technical papers that were widely cited in the AI research community, raising the profile of the company in academic circles.
- Participated in collaborative projects with universities, fostering innovation and sharing knowledge with emerging talents in AI.
- Served as a key speaker in several AI symposiums, presenting research findings and gaining industry recognition.
- Provided expert consultation on machine learning strategy to multiple startups, leading to successful product launches and funding rounds.
- Developed custom algorithms for clients, resulting in enhanced operational efficiencies and customer engagement metrics.
- Conducted workshops and training sessions for client teams on the application of deep learning techniques, elevating their skill levels.
- Analyzed large datasets to identify trends and patterns, delivering insights that informed business strategies.
- Built and maintained strong relationships with clients, fostering trust and contributing to subsequent project opportunities.
SKILLS & COMPETENCIES
Certainly! Here’s a list of 10 skills that are related to deep learning for a job position in that field:
Neural Network Architecture Design: Proficiency in designing and implementing various neural network architectures such as CNNs, RNNs, and Transformers.
Programming Proficiency: Expertise in programming languages commonly used in deep learning, particularly Python, along with libraries like TensorFlow, PyTorch, or Keras.
Data Preprocessing: Skills in data cleaning, normalization, augmentation, and feature engineering to prepare datasets for training deep learning models.
Hyperparameter Tuning: Ability to optimize models through hyperparameter tuning techniques to achieve better accuracy and performance.
Model Evaluation and Validation: Knowledge of evaluation metrics (e.g., accuracy, precision, recall, F1-score) and techniques for validating model performance.
GPU Programming: Understanding of parallel computing and experience with GPU utilization for speeding up deep learning tasks.
Deep Learning Frameworks: Familiarity with popular deep learning frameworks and tools, including TensorFlow, PyTorch, and MXNet.
Transfer Learning: Experience with transfer learning techniques and pre-trained models to expedite model training and improve results.
Understanding of ML Concepts: Solid grasp of fundamental machine learning concepts and algorithms that complement deep learning, such as supervised vs. unsupervised learning.
Deployment and Integration: Skills in deploying deep learning models into production environments and integrating them with web or mobile applications.
These skills will help individuals excel in deep learning roles and contribute effectively to projects and team objectives.
COURSES / CERTIFICATIONS
Here’s a list of certifications and course offerings related to deep learning that are valuable for job positions in this field:
Deep Learning Specialization
Offered by: Coursera (Andrew Ng)
Duration: 3 months (Approx. 11 hours/week)
Dates Available: Continuous enrollmentTensorFlow Developer Certificate
Offered by: TensorFlow
Duration: Self-paced (approximately 2-3 months recommended)
Certification Exam Dates: Continuous availabilityDeep Learning with PyTorch: A 60 Minute Blitz
Offered by: PyTorch (official documentation/tutorial)
Duration: 1 hour (self-paced)
Dates Available: Continuous availabilityMIT Deep Learning for Self-Driving Cars
Offered by: MIT OpenCourseWare
Duration: 5 weeks (Approx. 5-10 hours/week)
Dates Available: Continuous enrollmentNeural Networks and Deep Learning
Offered by: edX (University of Microsoft)
Duration: 6 weeks (Approx. 4-6 hours/week)
Dates Available: Continuous enrollment
These certifications and courses provide foundational to advanced knowledge in deep learning and are recognized within the industry.
EDUCATION
Here’s a list of education qualifications related to deep learning for a job position in this field:
Master of Science in Computer Science
- Specialization: Machine Learning and Deep Learning
- University: Stanford University
- Dates: September 2020 - June 2022
Bachelor of Science in Data Science
- University: University of California, Berkeley
- Dates: September 2016 - May 2020
This combination provides a strong foundational knowledge and specialized skills relevant to deep learning positions.
Certainly! Here are 19 important hard skills in the field of deep learning that professionals should possess:
Programming Languages (Python, R, etc.)
- Proficiency in programming languages such as Python and R is fundamental for deep learning practitioners. Python, in particular, is favored due to its simplicity and a vast array of libraries such as TensorFlow and PyTorch that facilitate deep learning projects.
Linear Algebra
- Understanding linear algebra is crucial for grasping the mathematical underpinnings of deep learning models. Concepts like vectors, matrices, and operations like dot products are frequently applied in algorithm design and optimization.
Calculus
- Calculus, especially differential calculus, is essential for optimizing deep learning models. It provides insights into gradient descent and how changes in variables affect outputs, enabling fine-tuning of algorithms.
Statistics and Probability
- Statistics and probability knowledge help in making informed decisions about data interpretation and model evaluation. Professionals must understand concepts like distributions, hypotheses testing, and confidence intervals to ascertain model reliability.
Neural Networks Architecture
- Deep understanding of various neural network architectures, including CNNs, RNNs, and GANs, is vital. Each architecture has unique applications, and knowing their strengths and weaknesses enables professionals to select and implement the best model for specific tasks.
Deep Learning Frameworks
- Familiarity with popular deep learning frameworks like TensorFlow, PyTorch, and Keras is essential for developing, training, and deploying models efficiently. Mastery of these tools enhances productivity and collaboration in data science teams.
Data Preprocessing
- Skills in data cleaning, normalization, and augmentation are crucial for preparing datasets for deep learning. Proper preprocessing ensures that models are trained on high-quality data, leading to better performance and generalization.
Model Evaluation and Validation
- Understanding various performance metrics (e.g., accuracy, precision, recall, F1-score) is key to evaluating deep learning models. Professionals must be adept at implementing cross-validation techniques to prevent overfitting and ensure model robustness.
Hyperparameter Tuning
- Mastery of hyperparameter tuning techniques, such as grid search and random search, is necessary for optimizing model performance. This skill involves systematically adjusting model parameters to enhance accuracy and efficiency.
Computer Vision
- For those specializing in computer vision tasks, knowledge of techniques such as image processing, feature extraction, and object detection is essential. Familiarity with landmark architectures like ResNet and YOLO can significantly propel a project’s success.
Natural Language Processing (NLP)
- Professionals working on NLP projects should be well-versed in techniques such as tokenization, stemming, and transformer models. Understanding how neural networks can process human language is crucial for developing applications like chatbots and automatic summarizers.
Reinforcement Learning
- Knowledge of reinforcement learning frameworks is important for developing algorithms that learn optimal behaviors through trial and error. This area is often used in gaming, robotics, and decision-making systems.
Version Control Systems (Git)
- Proficiency in version control systems like Git is necessary for maintaining code integrity and collaboration within teams. It enables tracking changes, reverting to previous states, and efficiently collaborating on shared codebases.
Cloud Computing and APIs
- Familiarity with cloud computing platforms such as AWS, Azure, or Google Cloud is crucial for deploying deep learning models at scale. Knowledge of API integration is also necessary for building applications that leverage deep learning capabilities.
Big Data Technologies
- Understanding big data tools such as Apache Hadoop and Apache Spark is essential for handling large datasets typically used in deep learning. This expertise allows professionals to process and analyze massive amounts of data efficiently.
Deployment Strategies
- Skills in deploying models into production environments and scaling them effectively are critical for real-world applications. Familiarity with containerization technologies like Docker and orchestration tools like Kubernetes enhances deployment capabilities.
Model Interpretability
- Knowledge of techniques for interpreting model predictions, such as SHAP and LIME, is essential for fostering trust in AI systems. Understanding why a model makes specific predictions helps in debugging and ensuring ethical AI usage.
Continuous Learning and Adaptation
- Staying updated with the latest advancements in deep learning is vital due to the field's rapid evolution. Commitment to lifelong learning through courses, blogs, and research papers is necessary for remaining competitive in the industry.
Collaboration and Communication Skills
- While not strictly a technical skill, the ability to collaborate and communicate effectively within multidisciplinary teams is crucial. Professionals must articulate complex ideas clearly to stakeholders and colleagues from different backgrounds.
Each of these skills contributes to the comprehensive knowledge required to excel in the deep learning field, ensuring professionals can effectively develop and deploy their models.
Job Position: Deep Learning Engineer
Top Hard Skills:
Proficiency in Programming Languages: Expertise in Python and C++, with a strong understanding of libraries such as TensorFlow, PyTorch, and Keras to implement deep learning models effectively.
Mathematics and Statistics: Strong foundation in linear algebra, calculus, probability, and statistics to understand and develop complex algorithms used in deep learning.
Neural Network Architecture: In-depth knowledge of various neural network architectures (CNNs, RNNs, GANs, etc.) and their applications in solving specific problems.
Data Preprocessing and Augmentation: Skills in data cleaning, preprocessing techniques, and augmentation methods to ensure high-quality input data for training models.
Model Optimization Techniques: Familiarity with techniques such as hyperparameter tuning, regularization, and optimization algorithms (SGD, Adam, etc.) to enhance model performance.
Experience with Cloud Computing: Proficient in utilizing cloud platforms (like AWS, Google Cloud, Azure) for scalable deep learning solutions and big data processing.
Deployment and Monitoring: Knowledge of deploying models in production environments using tools like Docker, Kubernetes, and monitoring performance using A/B testing and other evaluation metrics.
Generate Your Cover letter Summary with AI
Accelerate your Cover letter crafting with the AI Cover letter Builder. Create personalized Cover letter summaries in seconds.
Related Resumes:
Generate Your NEXT Resume with AI
Accelerate your Resume crafting with the AI Resume Builder. Create personalized Resume summaries in seconds.