Machine Learning Skills for Your Resume: 19 Essential Competencies
Sure! Here are six sample cover letters for various subpositions related to "machine learning," with the specified fields filled in for each.
### Sample 1
- **Position number:** 1
- **Position title:** Machine Learning Engineer
- **Position slug:** machine-learning-engineer
- **Name:** John
- **Surname:** Doe
- **Birthdate:** January 15, 1990
- **List of 5 companies:** Apple, Microsoft, Meta, NVIDIA, Amazon
- **Key competencies:** Python, TensorFlow, Neural Networks, Data Analysis, Model Deployment
**Cover Letter:**
---
[Your Address]
[City, State, Zip]
[Email]
[Phone Number]
[Date]
[Hiring Manager's Name]
[Company Name]
[Company Address]
[City, State, Zip]
Dear [Hiring Manager's Name],
I am writing to express my interest in the Machine Learning Engineer position at [Company Name]. With a solid foundation in machine learning, particularly in developing neural networks using TensorFlow, I believe I am a perfect fit for your innovative team.
I have had the opportunity to work with leading companies like Apple and Microsoft, where I honed my skills in Python and data analysis. My experience in model deployment has enabled me to create robust solutions that enhance performance across different applications. I am eager to bring my passion for machine learning to [Company Name] and contribute to your groundbreaking projects.
Thank you for considering my application. I look forward to the possibility of discussing my qualifications further.
Warm regards,
John Doe
---
### Sample 2
- **Position number:** 2
- **Position title:** Data Scientist
- **Position slug:** data-scientist
- **Name:** Jane
- **Surname:** Smith
- **Birthdate:** June 22, 1988
- **List of 5 companies:** Google, IBM, Salesforce, LinkedIn, Spotify
- **Key competencies:** R, Data Visualization, Machine Learning Algorithms, Feature Engineering, Statistical Analysis
**Cover Letter:**
---
[Your Address]
[City, State, Zip]
[Email]
[Phone Number]
[Date]
[Hiring Manager's Name]
[Company Name]
[Company Address]
[City, State, Zip]
Dear [Hiring Manager's Name],
I am excited to apply for the Data Scientist position at [Company Name]. With over five years of experience in leveraging machine learning algorithms to extract insight from large datasets, I am confident in my ability to contribute to your analytics team.
During my tenure at Google, I developed several feature engineering techniques that improved model accuracy significantly. My proficiency in R and data visualization has allowed me to present complex data insights clearly and concisely to stakeholders. I am passionate about using data to drive strategic decision-making and would love the opportunity to bring my skills to [Company Name].
Thank you for your time and consideration. I look forward to the possibility of collaborating with your talented team.
Best regards,
Jane Smith
---
### Sample 3
- **Position number:** 3
- **Position title:** Machine Learning Researcher
- **Position slug:** machine-learning-researcher
- **Name:** Alex
- **Surname:** Johnson
- **Birthdate:** March 10, 1985
- **List of 5 companies:** Stanford University, Google Research, Facebook AI Research, MIT, OpenAI
- **Key competencies:** Research Methodology, Deep Learning, Improvisation in Algorithms, Publish Academic Papers, Cross-disciplinary Collaboration
**Cover Letter:**
---
[Your Address]
[City, State, Zip]
[Email]
[Phone Number]
[Date]
[Hiring Manager's Name]
[Company Name]
[Company Address]
[City, State, Zip]
Dear [Hiring Manager's Name],
I am writing to express my interest in the Machine Learning Researcher position at [Company Name]. With a Ph.D. in Computer Science and extensive experience in deep learning research, I am keen to contribute to the innovative work being done in your organization.
My background includes tenure at renowned institutions such as Stanford University and MIT where I developed novel algorithms that have been published in leading academic journals. I thrive in cross-disciplinary environments, collaborating with diverse teams to push the boundaries of what is possible with machine learning.
I am eager to explore how I can contribute to the cutting-edge projects at [Company Name]. Thank you for considering my application.
Sincerely,
Alex Johnson
---
### Sample 4
- **Position number:** 4
- **Position title:** AI Developer
- **Position slug:** ai-developer
- **Name:** Emily
- **Surname:** Williams
- **Birthdate:** September 5, 1992
- **List of 5 companies:** Amazon, NVIDIA, Tesla, Adobe, Tencent
- **Key competencies:** Java, AI Frameworks, Custom Algorithm Design, Software Development Life Cycle (SDLC), Cloud Computing
**Cover Letter:**
---
[Your Address]
[City, State, Zip]
[Email]
[Phone Number]
[Date]
[Hiring Manager's Name]
[Company Name]
[Company Address]
[City, State, Zip]
Dear [Hiring Manager's Name],
I am eager to apply for the position of AI Developer at [Company Name]. With a rich background in software development and a specialization in AI frameworks, I am excited about the opportunity to develop innovative solutions that can drive your business forward.
Working at Amazon provided me with hands-on experience in Java and the software development life cycle. My involvement in cloud computing projects has also refined my skills in developing scalable solutions. I am passionate about leveraging AI to create transformative technologies and am thrilled at the prospect of contributing to [Company Name].
Thank you for your consideration. I hope to discuss my application with you soon.
Best,
Emily Williams
---
### Sample 5
- **Position number:** 5
- **Position title:** Machine Learning Product Manager
- **Position slug:** ml-product-manager
- **Name:** Michael
- **Surname:** Brown
- **Birthdate:** April 18, 1987
- **List of 5 companies:** Dropbox, HubSpot, Stripe, Oracle, Zoom
- **Key competencies:** Product Development, Data-Driven Decision Making, Agile Methodologies, Stakeholder Engagement, User Experience Design
**Cover Letter:**
---
[Your Address]
[City, State, Zip]
[Email]
[Phone Number]
[Date]
[Hiring Manager's Name]
[Company Name]
[Company Address]
[City, State, Zip]
Dear [Hiring Manager's Name],
I am excited to submit my application for the Machine Learning Product Manager position at [Company Name]. My background in product development combined with a solid understanding of machine learning concepts uniquely positions me to drive impactful product initiatives.
During my tenure at Dropbox, I successfully led a project that integrated machine learning algorithms into our product line, significantly enhancing user experiences. I thrive in agile environments and have proven expertise in stakeholder engagement, ensuring that product goals are met efficiently and effectively.
I would be thrilled to bring my expertise to [Company Name] and help develop products that harness the power of machine learning.
Thank you for your time and consideration. I look forward to the possibility of discussing this exciting opportunity with you.
Kind regards,
Michael Brown
---
### Sample 6
- **Position number:** 6
- **Position title:** Machine Learning Analyst
- **Position slug:** machine-learning-analyst
- **Name:** Sarah
- **Surname:** Taylor
- **Birthdate:** December 28, 1991
- **List of 5 companies:** Netflix, Airbnb, Uber, eBay, Shopify
- **Key competencies:** Data Mining, Predictive Modeling, SQL, Reporting, TensorFlow
**Cover Letter:**
---
[Your Address]
[City, State, Zip]
[Email]
[Phone Number]
[Date]
[Hiring Manager's Name]
[Company Name]
[Company Address]
[City, State, Zip]
Dear [Hiring Manager's Name],
I am writing to apply for the Machine Learning Analyst position at [Company Name]. With a strong analytical background and hands-on experience in predictive modeling and data mining, I am excited about the opportunity to contribute to your team.
In my previous role at Netflix, I developed reporting models that improved decision-making processes across departments. My proficiency in SQL and TensorFlow has enabled me to turn complex data into actionable insights effectively. I am passionate about using data to solve problems and drive strategic initiatives.
I would love the chance to discuss how my skill set can align with the goals at [Company Name]. Thank you for your consideration.
Sincerely,
Sarah Taylor
---
Feel free to customize each cover letter further to better fit your style or the specific company you are applying to!
Machine Learning: 19 Essential Skills to Boost Your Resume for Success
Why This Machine-Learning Skill is Important
In today’s data-driven world, mastering machine learning is essential for solving complex problems and making informed decisions across diverse fields. This skill empowers individuals to analyze vast datasets, uncover patterns, and generate predictions, leading to enhanced operational efficiency and innovative solutions. Industries such as healthcare, finance, and marketing leverage machine learning to optimize processes, improve customer experiences, and drive revenue growth. As organizations increasingly rely on data for strategic planning, the ability to harness machine learning becomes a competitive advantage.
Furthermore, as technology continues to evolve, the demand for machine learning expertise is skyrocketing. Professionals equipped with this skill not only open doors to lucrative career opportunities but also play a pivotal role in shaping the future of artificial intelligence. By understanding algorithms, model training, and data preprocessing, learners can contribute to advancements in automation, predictive analytics, and smart systems, ensuring they remain at the forefront of technological innovation.
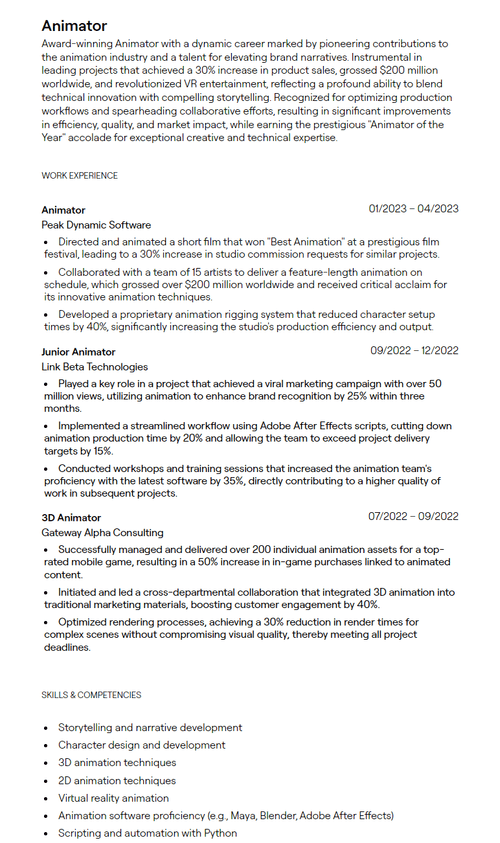
Machine learning is a pivotal skill in today's data-driven landscape, enabling the development of intelligent systems that can analyze vast amounts of data to make predictions and automate decisions. Success in this field demands talents in programming (especially Python and R), statistical analysis, and a strong understanding of algorithms. Additionally, problem-solving and critical thinking are essential for refining models and interpreting results. To secure a job in machine learning, candidates should pursue relevant coursework, engage in hands-on projects, build a robust portfolio, and network within the industry to showcase their expertise and commitment to continuous learning.
Sure! Here are ten key factors that are actually required for success in developing machine learning skills:
Strong Mathematics Foundation
A solid understanding of algebra, calculus, probability, and statistics is crucial. These mathematical concepts form the backbone of many algorithms and are essential for evaluating model performance.Programming Proficiency
Familiarity with programming languages such as Python or R is essential. Structured programming skills enable you to implement algorithms, manipulate data, and develop machine learning models effectively.Data Manipulation Skills
The ability to clean, preprocess, and manipulate data using libraries like Pandas or NumPy helps in transforming raw datasets into formats suitable for analysis. Good data handling lays the groundwork for effective model training and evaluation.Understanding Machine Learning Algorithms
Grasping core machine learning concepts, including supervised and unsupervised learning, model evaluation, and feature selection, will facilitate your ability to choose and apply appropriate algorithms for different tasks.Hands-On Experience
Practical experience through projects, coding challenges, or contributions to open-source projects can reinforce your theoretical understanding. Building and iterating real-world models helps solidify your skills while exposing you to the nuances of implementation.Familiarity with Tools and Libraries
Proficiency in tools like TensorFlow, PyTorch, or Scikit-learn can enhance your efficiency in building models. Understanding these libraries allows you to leverage pre-built functions and optimize your workflow.Data Visualization Skills
The ability to visualize data and model performance using libraries such as Matplotlib or Seaborn is important. Effective visualizations help communicate insights and identify patterns that may not be immediately apparent from raw data.Critical Thinking and Problem-Solving
Developing the ability to approach problems methodically and think critically about solutions is essential. Machine learning often involves troubleshooting and refining approaches, requiring a strong problem-solving mindset.Continuous Learning and Adaptability
The field of machine learning is rapidly evolving, so a commitment to lifelong learning and keeping up with new research, technologies, and methodologies is vital. Being adaptable will help you stay relevant and effective in your work.Collaboration and Communication
Working effectively within teams and communicating technical concepts to non-experts are key interpersonal skills. Collaboration fosters diverse ideas and can lead to better solutions, while good communication ensures that everyone involved understands the project's goals.
Sample Mastering Machine Learning: From Fundamentals to Advanced Applications skills resume section:
When crafting a resume that highlights machine learning skills, it's crucial to emphasize relevant technical competencies, such as proficiency in programming languages (e.g., Python, R) and libraries (e.g., TensorFlow, Scikit-learn). Detail hands-on experience with machine learning algorithms, data preprocessing, and model evaluation. Include specific projects or achievements that demonstrate your ability to apply these skills in real-world scenarios. Additionally, showcase collaboration in cross-functional teams, problem-solving abilities, and any contributions to open-source projects or publications. Certifications and continued education in machine learning techniques can also enhance credibility and appeal to potential employers.
WORK EXPERIENCE
SKILLS & COMPETENCIES
COURSES / CERTIFICATIONS
Here’s a list of five certifications and complete courses relevant to machine learning, along with their dates:
Machine Learning by Stanford University (Coursera)
- Duration: Approx. 11 weeks
- Date Completed: September 2022
Deep Learning Specialization by deeplearning.ai (Coursera)
- Duration: Approx. 3 months (5 courses)
- Date Completed: December 2022
Professional Certificate in Machine Learning and Artificial Intelligence by MIT (edX)
- Duration: 32 weeks
- Date Completed: June 2023
Applied Data Science with Python Specialization by the University of Michigan (Coursera)
- Duration: Approx. 5 months (5 courses)
- Date Completed: April 2023
Google Cloud Professional Machine Learning Engineer Certification
- Preparation Duration: 3 months
- Date Achieved: August 2023
EDUCATION
Here are 19 important hard skills that professionals in machine learning should possess, along with descriptions of each:
Programming Proficiency (Python, R, Java)
- Proficiency in programming languages like Python and R is essential for machine learning development. These languages offer robust libraries and frameworks, such as TensorFlow and scikit-learn, which facilitate algorithm implementation and data manipulation.
Mathematics and Statistics
- A strong foundation in mathematics and statistics is crucial for understanding algorithms and data models. Skills in linear algebra, calculus, probability, and inferential statistics help professionals evaluate data trends and derive insights from models.
Data Preprocessing and Cleaning
- Data is often messy and requires preprocessing before analysis. Skills in data cleaning, transformation, and normalization ensure that datasets are accurate and usable, which significantly affects the performance of machine learning models.
Machine Learning Algorithms
- In-depth knowledge of various machine learning algorithms, including supervised and unsupervised learning, is essential. Understanding when to apply techniques like regression, clustering, or decision trees can lead to better model performance and solution development.
Deep Learning Frameworks
- Familiarity with deep learning frameworks such as TensorFlow, Keras, and PyTorch is critical for working on complex models. These frameworks provide tools for building and training neural networks, which are fundamental in deep learning applications.
Feature Engineering
- The ability to extract and select features from raw data can significantly improve model accuracy. Feature engineering involves understanding data properties and creating meaningful variables that enhance the predictive power of models.
Model Evaluation and Validation
- Evaluating model performance is key to ensuring that machine learning solutions are reliable. Skills in metrics like accuracy, precision, recall, and F1 score, along with techniques like cross-validation, help in assessing and improving models.
Big Data Technologies
- Proficiency in big data technologies such as Hadoop, Spark, and Hive enables professionals to handle large volumes of data efficiently. Understanding these tools is essential for processing and analyzing datasets that exceed traditional storage and processing capabilities.
Data Visualization
- Skills in data visualization using tools like Matplotlib, Seaborn, or Tableau help communicate insights effectively. Visual representations of data can uncover patterns and trends that might be missed in raw data or text-based formats.
Cloud Computing
- Knowledge of cloud platforms such as AWS, Google Cloud, or Azure is increasingly important for deploying machine learning models. Cloud services offer scalable processing power and storage capabilities, facilitating comprehensive machine learning applications.
Reinforcement Learning
- Understanding reinforcement learning concepts is essential for developing adaptive models that learn from feedback. Skills in designing reward systems and understanding the exploration-exploitation trade-off are crucial for success in dynamic environments.
Natural Language Processing (NLP)
- Proficiency in NLP techniques enables professionals to work with text data effectively. Understanding language models, sentiment analysis, and text classification helps in building applications like chatbots and translation services.
Computer Vision Techniques
- Skills in computer vision are vital for projects involving image and video data. Knowledge of convolutional neural networks (CNNs) and image processing techniques allows professionals to create applications ranging from facial recognition to autonomous vehicles.
Algorithm Optimization
- The ability to optimize algorithms can improve model efficiency and execution speed. Skills in techniques like hyperparameter tuning and algorithm selection ensure that the best possible models are developed within practical constraints.
Time Series Analysis
- Time series analysis skills are important for applications dealing with temporal data. Proficiency in forecasting, trend detection, and seasonal decomposition helps professionals analyze and predict future values in time-dependent datasets.
Ethics and Bias in AI
- An understanding of ethics and bias in artificial intelligence is crucial for responsible machine learning practice. Professionals are tasked with ensuring that models are fair, transparent, and do not perpetuate societal biases.
Deployment and Monitoring
- Skills in model deployment and monitoring ensure that machine learning models can be successfully operationalized and assessed post-deployment. Knowledge of APIs, containers (e.g., Docker), and monitoring frameworks is essential for maintaining model performance over time.
Database Management
- Proficiency in SQL and NoSQL databases is necessary for data extraction and management. Skills in querying databases and understanding data structures support effective data retrieval and organization, leading to better analysis outcomes.
Collaboration and Version Control
- Familiarity with collaboration tools like Git is vital for version control and teamwork in machine learning projects. Being able to collaborate with others ensures that code is maintainable and that projects can scale efficiently with multiple contributors.
Each of these skills contributes to the overall competence of machine learning professionals, enabling them to tackle complex problems and create innovative solutions in various domains.
Generate Your Cover letter Summary with AI
Accelerate your Cover letter crafting with the AI Cover letter Builder. Create personalized Cover letter summaries in seconds.
Related Resumes:
Generate Your NEXT Resume with AI
Accelerate your Resume crafting with the AI Resume Builder. Create personalized Resume summaries in seconds.