null
Here are six sample cover letters for positions related to statistical modeling. The details have been filled in as requested:
### Sample 1
**Position number:** 1
**Position title:** Statistical Analyst
**Position slug:** statistical-analyst
**Name:** Sarah
**Surname:** Thompson
**Birthdate:** 1990-07-15
**List of 5 companies:** Apple, Dell, Google, IBM, Microsoft
**Key competencies:** Statistical analysis, Data visualization, R programming, Predictive modeling, Machine learning
**Cover Letter:**
[Your Address]
[City, State, Zip]
[Email Address]
[Phone Number]
[Date]
[Hiring Manager’s Name]
[Company Name]
[Company Address]
[City, State, Zip]
Dear [Hiring Manager’s Name],
I am writing to apply for the Statistical Analyst position at [Company Name] as advertised. With a Master’s degree in Statistics and over four years of experience in data analysis and statistical modeling, I am excited about the opportunity to contribute to your team.
At my current position with [Your Current Company], I successfully developed predictive models that increased operational efficiency by 20%. My expertise in R programming and data visualization tools has allowed me to present complex data in an easily understandable format for stakeholders. I am particularly drawn to [Company’s Project/Initiative] and would love to apply my skills to support it.
I look forward to the opportunity to discuss how my background, skills, and enthusiasms can fit into the innovative projects at [Company Name]. Thank you for considering my application.
Sincerely,
Sarah Thompson
---
### Sample 2
**Position number:** 2
**Position title:** Data Scientist
**Position slug:** data-scientist
**Name:** Michael
**Surname:** Robinson
**Birthdate:** 1988-01-22
**List of 5 companies:** Google, IBM, Netflix, Adobe, Amazon
**Key competencies:** Machine learning, Statistical programming, Big data analysis, Python, Data storytelling
**Cover Letter:**
[Your Address]
[City, State, Zip]
[Email Address]
[Phone Number]
[Date]
[Hiring Manager’s Name]
[Company Name]
[Company Address]
[City, State, Zip]
Dear [Hiring Manager’s Name],
I am excited to apply for the Data Scientist position at [Company Name]. With a strong foundation in statistical modeling and extensive experience in machine learning, I am well-prepared to take on the challenges at your company.
During my time with [Your Previous Company], I enhanced our data-driven decision-making processes and developed algorithms that improved project outputs by 30%. My proficiency in Python and big data technologies aligns with your requirements, and I thrive on translating complex datasets into actionable insights.
I am eager to bring my expertise and passion for data science to the talented team at [Company Name]. Thank you for considering my application, and I hope to discuss my fit for this role further.
Best regards,
Michael Robinson
---
### Sample 3
**Position number:** 3
**Position title:** Quantitative Analyst
**Position slug:** quantitative-analyst
**Name:** Jessica
**Surname:** Allen
**Birthdate:** 1995-04-10
**List of 5 companies:** Goldman Sachs, JPMorgan Chase, Morgan Stanley, Citadel, BlackRock
**Key competencies:** Financial modeling, Statistical techniques, Risk assessment, Matlab, SQL
**Cover Letter:**
[Your Address]
[City, State, Zip]
[Email Address]
[Phone Number]
[Date]
[Hiring Manager’s Name]
[Company Name]
[Company Address]
[City, State, Zip]
Dear [Hiring Manager’s Name],
I am writing to express my interest in the Quantitative Analyst position at [Company Name]. With my academic background in Financial Engineering and two years of experience in quantitative analysis, I am well-versed in statistical modeling techniques critical for investment management.
At [Your Current Company], I developed financial models that successfully predicted market trends and minimized risk. My expertise in Matlab and SQL allowed my team to efficiently process and analyze vast datasets, leading to timely and strategic investment decisions.
I am enthusiastic about the chance to bring my dedication and analytical skills to [Company Name]. Thank you for considering my application.
Sincerely,
Jessica Allen
---
### Sample 4
**Position number:** 4
**Position title:** Biostatistician
**Position slug:** biostatistician
**Name:** David
**Surname:** Carter
**Birthdate:** 1985-11-30
**List of 5 companies:** Pfizer, Johnson & Johnson, Merck, Novartis, GSK
**Key competencies:** Clinical trials, Statistical software (SAS, R), Data analysis, Hypothesis testing, Epidemiological studies
**Cover Letter:**
[Your Address]
[City, State, Zip]
[Email Address]
[Phone Number]
[Date]
[Hiring Manager’s Name]
[Company Name]
[Company Address]
[City, State, Zip]
Dear [Hiring Manager’s Name],
I am eager to apply for the Biostatistician position at [Company Name]. With over five years of experience in statistical modeling for clinical research and a Ph.D. in Biostatistics, I am confident in my ability to contribute meaningfully to your team.
During my tenure at [Your Current Company], I effectively led statistical analyses for multiple clinical trials, helping to streamline processes and improve outcomes. My skills in SAS and R are complemented by a solid understanding of epidemiological principles and methodologies.
I am excited about the potential to collaborate with [Company Name] on groundbreaking research, and I look forward to the opportunity to discuss how my skills align with your goals.
Thank you for your consideration.
Best,
David Carter
---
### Sample 5
**Position number:** 5
**Position title:** Research Analyst
**Position slug:** research-analyst
**Name:** Emily
**Surname:** Martin
**Birthdate:** 1992-09-05
**List of 5 companies:** Bloomberg, The Economist, RAND Corporation, Pew Research Center, McKinsey & Company
**Key competencies:** Data interpretation, Statistical analysis, Report writing, SPSS, Tableau
**Cover Letter:**
[Your Address]
[City, State, Zip]
[Email Address]
[Phone Number]
[Date]
[Hiring Manager’s Name]
[Company Name]
[Company Address]
[City, State, Zip]
Dear [Hiring Manager’s Name],
I am thrilled to apply for the Research Analyst position at [Company Name]. With a solid background in statistical analysis and data interpretation, combined with my passion for evidence-based research, I am excited about the possibility of joining your team.
At [Your Current Company], I have produced in-depth reports that have guided strategic decisions, utilizing SPSS and Tableau for data visualization to effectively communicate complex findings to diverse audiences. My experience in economic and social research aligns well with the projects at [Company Name].
Thank you for considering my application. I would love the opportunity to discuss my qualifications in further detail.
Best regards,
Emily Martin
---
### Sample 6
**Position number:** 6
**Position title:** Machine Learning Engineer
**Position slug:** machine-learning-engineer
**Name:** Robert
**Surname:** Wilson
**Birthdate:** 1989-03-12
**List of 5 companies:** Facebook, Tesla, NVIDIA, Oracle, Salesforce
**Key competencies:** Machine learning algorithms, Data mining, Python, TensorFlow, Data preprocessing
**Cover Letter:**
[Your Address]
[City, State, Zip]
[Email Address]
[Phone Number]
[Date]
[Hiring Manager’s Name]
[Company Name]
[Company Address]
[City, State, Zip]
Dear [Hiring Manager’s Name],
I am interested in the Machine Learning Engineer position at [Company Name]. With over five years of experience in building robust machine learning models and a solid foundation in statistical modeling, I am confident in my ability to contribute to your innovative projects.
In my previous role at [Your Current Company], I designed and implemented machine learning algorithms that significantly enhanced predictive accuracy. My proficiency in Python and TensorFlow has allowed me to efficiently handle complex data sets and deliver actionable insights.
I look forward to bringing my expertise in machine learning to [Company Name] and contributing to your team's success. Thank you for considering my application.
Sincerely,
Robert Wilson
---
Feel free to adjust any of the details to better fit your needs or preferences!
null
Why This Statistical-Modeling Skill is Important
Statistical modeling is an essential skill that empowers individuals and organizations to make informed decisions based on data analysis. By employing various statistical techniques, one can uncover patterns, relationships, and trends within complex datasets. This skill not only enhances the ability to predict future outcomes but also enables effective communication of insights derived from data, thereby facilitating strategic planning and risk management. In today's data-driven world, businesses that harness statistical modeling can gain a competitive edge by optimizing operations and improving customer satisfaction through targeted solutions.
Moreover, statistical modeling plays a crucial role in fields such as healthcare, finance, and marketing, where decision-making needs to be grounded in empirical evidence. As organizations face increasing amounts of data, the capability to construct robust models becomes vital for identifying key variables and understanding their impacts on overall performance. Ultimately, proficiency in statistical modeling transforms raw data into valuable information, fostering innovation and driving growth in various sectors.
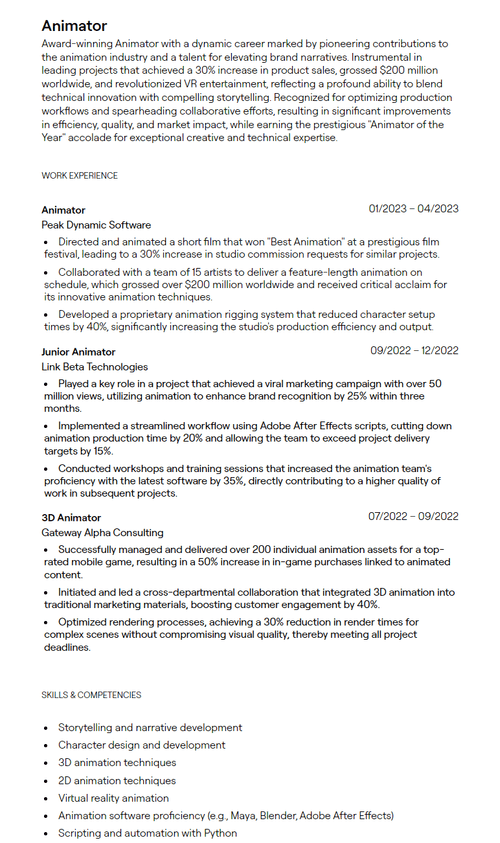
null
Sure! Here are 10 bullet points highlighting what is actually required for success in statistical modeling skills:
Strong Mathematical Foundation
A solid understanding of mathematics, particularly calculus, linear algebra, and probability theory, is essential for comprehending statistical concepts and their applications in modeling.Programming Proficiency
Familiarity with programming languages such as R, Python, or Julia is crucial for implementing statistical models, performing data manipulation, and automating analyses.Data Preprocessing Skills
Ability to clean and preprocess data — including handling missing values, normalization, and transformation — is vital to ensure that the data is ready for accurate modeling.Understanding of Statistical Methods
Knowledge of a range of statistical techniques (e.g., regression, hypothesis testing, Bayesian statistics) is required to choose the appropriate method for specific modeling tasks.Domain Knowledge
Having a good grasp of the subject area you are working in enables you to apply statistical models more effectively and provide insights relevant to the context of the data.Experience with Data Visualization
Skillful data visualization helps in understanding data distributions and relationships, which can inform model selection and enhance communication of results to stakeholders.Model Evaluation Techniques
Understanding how to assess model performance using metrics like accuracy, precision, and recall, as well as techniques like cross-validation, is crucial for ensuring robust models.Iterative Problem-Solving Mindset
Statistical modeling often requires revisiting and refining models based on findings and feedback. A willingness to iterate can lead to more effective solutions and insights.Collaboration and Communication Skills
Being able to work in teams and communicate complex findings clearly to non-experts is essential, as models need to be understandable and actionable for decision-makers.Continuous Learning and Adaptability
The field of statistical modeling is always evolving. Staying updated with new methods, software, and best practices through continuous education ensures that your skills remain relevant and effective.
Sample null skills resume section:
null
null
WORK EXPERIENCE
null
SKILLS & COMPETENCIES
Here are 10 skills related to the main statistical modeling skill for a job position:
- Data Analysis: Proficiency in analyzing datasets to extract meaningful insights.
- Statistical Software Proficiency: Experience with software such as R, Python, SAS, or SPSS for statistical modeling.
- Quantitative Research: Ability to design and conduct quantitative research studies and experiments.
- Machine Learning: Knowledge of machine learning techniques and algorithms to enhance statistical models.
- Data Visualization: Skills in creating visual representations of data using tools like Tableau, Matplotlib, or ggplot.
- Hypothesis Testing: Understanding of inferential statistics, including hypothesis testing and confidence intervals.
- Regression Analysis: Expertise in various regression techniques (linear, logistic, etc.) for predictive modeling.
- Data Cleaning and Preparation: Proficient in preprocessing and cleaning data for accurate analysis and modeling.
- Statistical Reporting: Ability to communicate statistical findings effectively through reports and presentations.
- Programming Skills: Familiarity with programming languages (e.g., Python, R) for coding statistical models and simulations.
COURSES / CERTIFICATIONS
null
EDUCATION
Here’s a list of educational qualifications related to statistical modeling:
Master of Science in Statistics
- Institution: University of California, Berkeley
- Dates: August 2019 - May 2021
Bachelor of Science in Mathematics
- Institution: University of Michigan, Ann Arbor
- Dates: September 2015 - May 2019
This educational background is typically relevant for positions requiring statistical modeling skills.
Here are 19 important hard skills that professionals in statistical modeling should possess, each accompanied by a brief description:
Statistical Analysis
- Proficient statistical analysis involves the use of statistical methods to summarize and interpret data. Analysts must be skilled in hypothesis testing, regression analysis, and variance analysis to extract meaningful insights from data sets.
Data Manipulation
- This skill encompasses cleaning, transforming, and organizing data to make it suitable for analysis. Familiarity with tools like SQL or Python libraries (such as pandas) is essential to efficiently handle and prepare large datasets.
Machine Learning Algorithms
- Understanding core machine learning algorithms, such as linear regression, decision trees, and neural networks, is vital. Professionals should be able to select and apply the appropriate algorithm to solve specific problems based on data characteristics.
Statistical Software Proficiency
- Mastery of statistical software tools like R, SAS, SPSS, or Python’s statistical libraries (SciPy, StatsModels) is crucial. These tools enable practitioners to conduct complex analyses and model building effectively.
Data Visualization
- Creating clear and informative visual representations of data is important for communicating findings. Skills in tools like Tableau, Matplotlib, or ggplot allow professionals to illustrate trends and analytical results compellingly.
Experimental Design
- Knowledge of how to plan and execute experiments is critical for statistical modeling. This includes the ability to define objectives, select appropriate methods, and implement randomization techniques to obtain reliable results.
Sampling Techniques
- Understanding different sampling methods and their implications on data collection is essential. Professionals must be able to choose between random, stratified, or cluster sampling to ensure representativeness and minimize bias in their analyses.
Predictive Modeling
- This skill involves using historical data to forecast future outcomes. Proficiency in techniques like time-series analysis and regression models allows statisticians to build robust predictive models for decision-making.
Resampling Methods
- Familiarity with techniques such as bootstrapping and cross-validation helps in assessing the reliability of statistical estimates. These methods provide insights into model performance and variance.
Statistical Inference
- The ability to draw conclusions about populations based on samples is fundamental. Professionals must understand concepts such as confidence intervals and significance testing to make informed inferences.
Multivariate Analysis
- Knowledge of multivariate techniques, including factor analysis and cluster analysis, is necessary for analyzing more complex data sets. This skill helps in understanding the relationships between multiple variables simultaneously.
Time Series Analysis
- Proficiency in analyzing time-dependent data is key for sectors like finance and economics. Familiarity with ARIMA models, seasonal decomposition, and forecasting methodologies is crucial for effective temporal analyses.
Bayesian Statistics
- Understanding Bayesian concepts allows professionals to apply prior knowledge to current data. This approach enhances decision-making under uncertainty, offering a flexible framework for statistical modeling.
Quality Control and Improvement
- Skills in quality control techniques such as Six Sigma or control charts help in monitoring and improving processes. Statistical process control is crucial for maintaining data integrity and quality.
Ethical Data Handling
- Knowledge of ethical guidelines and regulations for handling data is essential. Professionals should be well-versed in best practices related to data privacy, confidentiality, and integrity to build trust and compliance.
Dimensionality Reduction
- Techniques such as PCA (Principal Component Analysis) or t-SNE (t-distributed Stochastic Neighbor Embedding) help in simplifying data representation. This skill is important for enhancing model efficiency and interpretability.
Feature Engineering
- The ability to create new features from raw data can significantly improve model performance. This requires creativity and domain knowledge to identify useful attributes that can enhance predictive capability.
Linear and Nonlinear Programming
- Proficiency in optimization techniques is important for solving problems in resource allocation and decision-making. Understanding linear and nonlinear programming helps in finding optimal solutions to complex issues.
Report Writing and Communication
- The ability to document findings clearly and cohesively is crucial. Strong report-writing skills enhance the communication of complex statistical insights to non-technical stakeholders, ensuring broad comprehension and application of results.
These hard skills form the foundation of a proficient statistical modeling professional, allowing them to navigate complex datasets, derive insights, and drive data-informed decision-making across various industries.
Generate Your Cover letter Summary with AI
Accelerate your Cover letter crafting with the AI Cover letter Builder. Create personalized Cover letter summaries in seconds.
Related Resumes:
Generate Your NEXT Resume with AI
Accelerate your Resume crafting with the AI Resume Builder. Create personalized Resume summaries in seconds.