Statistical Software Skills to Boost Your Resume: Unlock Career Growth
Sure, here are six sample cover letters for subpositions related to "statistical-software", each with filled-in fields according to your request.
---
### Sample 1
**Position number:** 1
**Position title:** Statistical Software Developer
**Position slug:** statistical-software-developer
**Name:** John
**Surname:** Doe
**Birthdate:** January 15, 1990
**List of 5 companies:** IBM, Microsoft, Oracle, SAS, RStudio
**Key competencies:** Proficient in R and Python, experience with machine learning algorithms, strong problem-solving skills, familiarity with data visualization tools, excellent teamwork capabilities.
---
Dear Hiring Manager,
I am writing to express my interest in the Statistical Software Developer position listed on your company’s website. With a robust background in data science and statistical programming, I am excited about the opportunity to contribute to your innovative team.
At my previous role with IBM, I successfully developed statistical software to analyze complex datasets, improving data processing time by 30%. My proficiency in R and Python allows me to efficiently implement advanced machine learning algorithms that drive insights and strategic decision-making. Furthermore, my experience working collaboratively within teams ensures that all perspectives are integrated into project approaches.
I am highly motivated to leverage my skills to design top-tier software solutions at your company. I look forward to the possibility of discussing my application further.
Thank you for considering my application.
Best regards,
John Doe
---
### Sample 2
**Position number:** 2
**Position title:** Statistical Analyst
**Position slug:** statistical-analyst
**Name:** Jane
**Surname:** Smith
**Birthdate:** March 22, 1985
**List of 5 companies:** Google, Amazon, Tableau, SAP, Minitab
**Key competencies:** Advanced knowledge of statistical models, expertise in data mining, proficient in SQL and Excel, strong analytical thinking, ability to communicate technical concepts to non-technical audiences.
---
Dear Hiring Manager,
I am very interested in the Statistical Analyst position at your esteemed company, as advertised on your careers page. My extensive background in data analysis and statistical modeling aligns well with the qualifications required for this role.
At Tableau, I honed my skills in data mining and advanced statistical techniques to derive insights from large datasets, which resulted in data-driven decision-making for clients. My proficiency in SQL allows me to manipulate data effectively, while my strong analytical thinking ensures comprehensive analyses that meet and exceed expectations.
I am eager to bring my analytical skills and passion for data to your team. Thank you for considering my application, and I look forward to the opportunity to discuss my fit for the position.
Warm regards,
Jane Smith
---
### Sample 3
**Position number:** 3
**Position title:** Data Scientist
**Position slug:** data-scientist
**Name:** Michael
**Surname:** Johnson
**Birthdate:** July 18, 1992
**List of 5 companies:** Facebook, LinkedIn, Spotify, SAS, Alteryx
**Key competencies:** Strong statistical analysis skills, expertise in machine learning frameworks, experience with Python and R programming, knowledge of big data technologies, effective communication skills.
---
Dear Hiring Manager,
I am excited to submit my application for the Data Scientist position at your company. With a solid foundation in statistical analysis and a strong passion for machine learning, I am eager to contribute to your data-driven initiatives.
During my tenure at Facebook, I developed predictive models using large datasets that improved user engagement by 15%. My expertise in Python and R, coupled with my knowledge of big data technologies like Hadoop, allows me to craft innovative solutions tailored to your analytics challenges.
I believe my unique skills and experience make me a great fit for this role, and I look forward to the chance to discuss how I can contribute to your team.
Sincerely,
Michael Johnson
---
### Sample 4
**Position number:** 4
**Position title:** Quantitative Analyst
**Position slug:** quantitative-analyst
**Name:** Emily
**Surname:** Davis
**Birthdate:** February 10, 1988
**List of 5 companies:** Deutsche Bank, JPMorgan, Goldman Sachs, Bloomberg, RiskMetrics
**Key competencies:** Strong background in quantitative finance, experience with statistical software tools, adept at financial modeling, effective project management skills, solid understanding of economic principles.
---
Dear Hiring Manager,
I am writing to express my interest in the Quantitative Analyst position at your firm. With a strong background in quantitative finance and proven experience in statistical software, I am confident in my ability to contribute to your team’s financial analysis initiatives.
At JPMorgan, I was responsible for developing models that analyzed market trends and risk factors, providing actionable insights to our trading desks. My experience working with various statistical software tools allows me to execute complex analyses while effectively managing multiple projects.
I am eager to apply my analytical skills to help your firm achieve its financial goals. Thank you for considering my application; I look forward to further discussing my qualifications.
Best,
Emily Davis
---
### Sample 5
**Position number:** 5
**Position title:** Research Scientist
**Position slug:** research-scientist
**Name:** David
**Surname:** Wilson
**Birthdate:** May 1, 1995
**List of 5 companies:** Pfizer, Novartis, AstraZeneca, Merck, GSK
**Key competencies:** Proficient in statistical programming, experience in experimental design, strong critical thinking abilities, knowledge with clinical trial data analysis, effective collaboration within multidisciplinary teams.
---
Dear Hiring Manager,
I am thrilled to apply for the Research Scientist position at your organization. With extensive experience in statistical programming and a strong foundation in experimental design, I am excited about the opportunity to contribute to your research projects.
In my role at Pfizer, I analyzed clinical trial data that significantly impacted drug development timelines. My proficiency in R and SAS ensures that my analyses are accurate and meaningful. I enjoy collaborating with multidisciplinary teams and sharing insights that drive scientific advancement.
I look forward to the opportunity to discuss how I can contribute to your esteemed organization and help innovate in the pharmaceutical field.
Kind regards,
David Wilson
---
### Sample 6
**Position number:** 6
**Position title:** Biostatistician
**Position slug:** biostatistician
**Name:** Sarah
**Surname:** Brown
**Birthdate:** November 30, 1987
**List of 5 companies:** Genentech, Amgen, Eli Lilly, Biogen, Regeneron
**Key competencies:** Expertise in biostatistical methodologies, experience with clinical data analysis, proficient in SAS and R, strong communication skills, ability to work under tight deadlines.
---
Dear Hiring Manager,
I am writing to express my interest in the Biostatistician position at your company. I have a solid background in biostatistical methodologies and a commitment to applying innovative statistical approaches to healthcare challenges.
At Genentech, I played a key role in analyzing clinical data, leading to significant breakthroughs in treatment efficacy. My proficiency in both SAS and R allows me to tackle complex datasets, while my strong communication skills enable me to effectively present my findings to various stakeholders.
I am eager to bring my skills in biostatistics to your organization and contribute to groundbreaking health research. Thank you for your consideration, and I hope to discuss my application further.
Sincerely,
Sarah Brown
---
Feel free to modify any details or let me know if you need more samples!
Statistical Software Skills: 19 Essential Skills for Your Resume Analytics
Why This Statistical-Software Skill Is Important
In today's data-driven world, proficiency in statistical software is essential for making informed decisions across industries. With the growing reliance on data analysis to drive business strategies, having the ability to manipulate, visualize, and interpret data through statistical software like R, Python, or SAS empowers professionals to uncover insights that would otherwise remain hidden. This skill not only enhances the accuracy of analysis but also fosters creativity in problem-solving, allowing users to explore data from various perspectives and derive actionable conclusions.
Furthermore, as organizations collect vast amounts of data, the demand for individuals skilled in statistical software continues to rise. Mastering these tools can elevate one's career prospects, making candidates more competitive in the job market. Whether it's for academic research, market analysis, or operational efficiency, the ability to harness statistical software is crucial for anyone looking to advance their professional skills in a world increasingly governed by data.
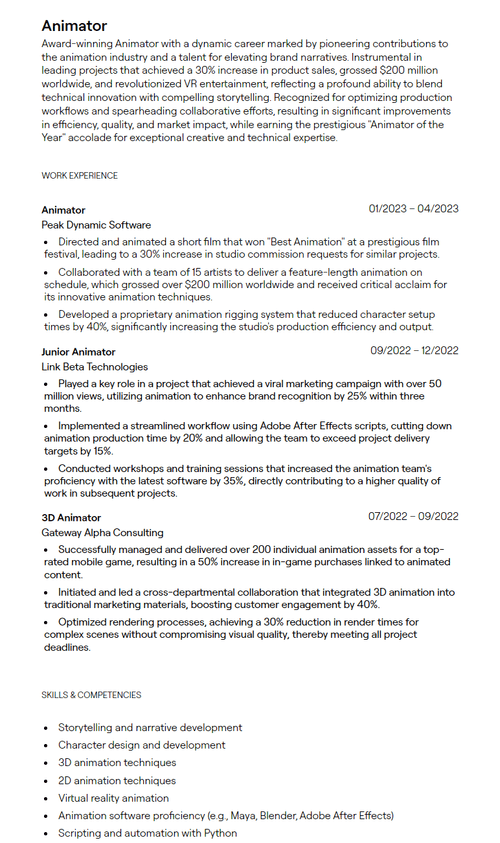
Proficiency in statistical software is critical in the data-driven landscape, enabling professionals to analyze complex datasets and derive actionable insights. This skill demands a strong analytical mindset, attention to detail, and familiarity with tools like R, Python, or SAS. Candidates should showcase their ability to interpret statistical results and communicate findings effectively. To secure a job in this field, gain hands-on experience through internships or projects, obtain relevant certifications, and build a portfolio demonstrating your analytical capabilities. Networking with industry professionals and staying updated on trends will further enhance your chances of landing a role in this competitive domain.
Statistical Software Proficiency: What is Actually Required for Success?
Certainly! Here are ten key points that outline what is actually required for success with statistical software skills:
1. **Understanding of Statistical Concepts**
A solid foundation in statistics is essential for effectively using statistical software. This includes knowledge of concepts such as probability distributions, hypothesis testing, and regression analysis, which allow for more accurate and insightful data interpretation.
2. **Familiarity with Relevant Software Tools**
Proficiency in widely-used statistical software, such as R, Python (with libraries like Pandas and SciPy), SAS, or SPSS, is crucial. Each tool has its unique features and syntax, so hands-on experience with the software is necessary to become efficient.
3. **Data Wrangling Skills**
The ability to clean, manipulate, and prepare data for analysis is a critical skill set. Data often comes in messy formats, and knowing how to handle missing values, outliers, and data types is vital for accurate analysis.
4. **Analytical Thinking**
Strong analytical skills help in interpreting data results and making data-driven decisions. This involves critically evaluating outputs, recognizing patterns, and formulating hypotheses based on the analysis.
5. **Coding Skills**
Basic programming skills, especially in languages like R and Python, are necessary to automate analyses and create custom functions. Proficiency in coding can enhance the efficiency and capability of statistical analyses far beyond what point-and-click software can offer.
6. **Attention to Detail**
Precision is key when working with data and statistical analyses. Small errors in data entry, programming code, or assumptions can lead to significant misinterpretations, so meticulousness is paramount in this line of work.
7. **Ability to Communicate Findings**
Success in statistical analysis is often tied to the ability to convey complex results to non-technical audiences. Effective communication skills, including visualization and presentation of data findings, are essential for influencing decisions and driving initiatives.
8. **Continuous Learning & Adaptation**
The field of statistics and data science is always evolving. Keeping up with the latest methodologies, software updates, and best practices through ongoing education and self-study enables one to remain effective and competitive in the field.
9. **Interdisciplinary Collaboration**
Collaborating with professionals from various fields (e.g., marketing, healthcare, finance) can enhance understanding and relevance of analyses. Being able to work well in teams and understand diverse perspectives leads to more impactful outcomes.
10. **Problem-Solving Mindset**
A strong problem-solving mindset is necessary for correctly identifying issues within data and generating actionable insights. This involves not only applying statistical tests but also knowing when to take a step back and reassess the approach based on observed results.
These skills form a solid framework for anyone looking to succeed in the field of statistical software and data analysis, making them both proficient practitioners and valuable contributors to their organizations.
Sample Mastering Statistical Software for Data Analysis skills resume section:
When crafting a resume highlighting statistical-software skills, emphasize proficiency in key software tools, showcasing your ability to analyze data and generate insights. Include specific examples of projects where you applied these skills effectively. Highlight your understanding of statistical concepts and methodologies, as well as any relevant certifications or training. Mention your experience with data visualization and reporting, emphasizing how you communicated findings to stakeholders. Tailor your resume to the job description, using industry-specific terminology and focusing on measurable outcomes that demonstrate your impact. Lastly, ensure clarity and organization for easy readability and comprehension.
• • •
We are seeking a skilled data analyst with expertise in statistical software such as R, SAS, or SPSS. The ideal candidate will analyze complex datasets, derive actionable insights, and support decision-making processes. Key responsibilities include data cleaning, statistical modeling, and presenting findings to stakeholders. Strong knowledge of hypothesis testing, regression analysis, and data visualization techniques is essential. The candidate should possess excellent problem-solving skills, attention to detail, and the ability to communicate complex concepts clearly. A degree in statistics, mathematics, or a related field is preferred. Join our dynamic team to drive data-driven strategies and enhance business performance.
WORK EXPERIENCE
- Led a cross-functional team to implement a new statistical forecasting model, resulting in a 30% increase in product sales over the following year.
- Developed and executed A/B testing strategies to optimize marketing campaigns, contributing to a global revenue growth of 15%.
- Presented data-driven insights to executive leadership, effectively influencing product development and strategic direction.
- Received the 'Excellence in Analytics' award for outstanding contributions to data analysis and visualization.
- Facilitated workshops on statistical software techniques, enhancing team proficiency and fostering a culture of continuous learning.
- Developed predictive models leveraging machine learning techniques, which improved customer retention rates by 20%.
- Collaborated with marketing teams to create comprehensive data reports that drove targeted campaigns and maximized ROI.
- Automated data collection and preprocessing tasks, reducing analysis time by 40% and improving workflow efficiency.
- Pioneered interactive dashboards using statistical software, facilitating real-time decision-making for project stakeholders.
- Mentored junior analysts, enhancing their skills in statistical software and fostering collaboration within the team.
- Analyzed market trends and consumer data, providing actionable insights that drove a 25% increase in sales within targeted demographics.
- Worked closely with software developers to design and implement an internal analytics platform, streamlining data analysis processes.
- Created comprehensive reports that highlighted key performance indicators, assisting in strategic quarterly planning.
- Trained team members on the usage of statistical software to enhance reporting capabilities and analytical skills.
- Conducted qualitative research to complement quantitative findings, presenting a well-rounded view of market dynamics.
- Supported the analysis of customer feedback data, helping inform product improvements that led to a 10% increase in satisfaction scores.
- Assisted senior analysts in conducting statistical tests and interpreting results to guide strategic initiatives.
- Became proficient in statistical software and contributed to the development of training materials for new hires.
- Compiled weekly performance reports for sales teams, providing them with insights to adjust strategies promptly.
- Actively participated in brainstorming sessions, contributing ideas that enhanced team collaboration and project outcomes.
SKILLS & COMPETENCIES
Certainly! Here’s a list of 10 skills related to a job position that emphasizes expertise in statistical software:
- Proficiency in statistical software (e.g., R, SAS, SPSS, or Python with libraries like pandas and NumPy)
- Data cleaning and preprocessing techniques
- Advanced statistical analysis (e.g., regression, ANOVA, multivariate analysis)
- Strong understanding of data visualization tools (e.g., Tableau, ggplot2, or Matplotlib)
- Ability to interpret and communicate statistical results effectively
- Knowledge of machine learning algorithms and their applications
- Familiarity with database management and querying languages (e.g., SQL)
- Experience with programming for automation and efficiency (e.g., using macros or scripting)
- Capability to design and conduct experiments or surveys
- Understanding of data ethics and compliance with relevant regulations (e.g., GDPR, HIPAA)
These skills highlight both technical proficiency and the analytical capabilities that are often required in positions focused on statistical software.
COURSES / CERTIFICATIONS
Here’s a list of five certifications and courses related to statistical software skills, along with their completion dates:
- **Coursera: Data Science Specialization (Johns Hopkins University)**
- Date Completed: June 2022
- **edX: Professional Certificate in Data Analysis (Microsoft)**
- Date Completed: February 2023
- **Udacity: Data Analyst Nanodegree**
- Date Completed: August 2023
- **SAS: SAS Certified Specialist: Base Programming Using SAS 9.4**
- Date Completed: January 2023
- **Google Data Analytics Professional Certificate**
- Date Completed: March 2023
These certifications and courses will enhance your skills in statistical software and data analysis, making you more competitive in related job positions.
EDUCATION
Here are a couple of educational qualifications related to a job position that emphasizes skills in statistical software:
- **Master of Science in Statistics**
- University of XYZ, Graduated May 2021
- Focused on advanced statistical analysis and data manipulation using software such as R and SAS.
- **Bachelor of Science in Data Science**
- University of ABC, Graduated May 2019
- Included coursework in statistical modeling and utilized software such as SPSS and Python for data analysis.
1. **R Programming**
- R is a powerful language used for statistical computing and graphics. Mastery of R enables professionals to perform complex data analysis, create visualizations, and develop custom statistical models.
2. **Python for Data Analysis**
- Python, with libraries such as Pandas and NumPy, is widely used for data manipulation and analysis. Proficiency in Python allows professionals to automate tasks and analyze large datasets efficiently.
3. **SAS (Statistical Analysis System)**
- SAS is a software suite used for advanced analytics, multivariate analysis, and predictive analytics. Given its strong presence in industries such as healthcare and finance, knowledge of SAS is essential for generating detailed reports and forecasts.
4. **SPSS (Statistical Package for the Social Sciences)**
- SPSS is particularly popular in social science research for its user-friendly interface and robust statistical capabilities. Professionals familiar with SPSS can easily conduct data analysis and interpret results using various statistical tests.
5. **MATLAB**
- MATLAB is often used for mathematical modeling, algorithm development, and data visualization. Proficiency in MATLAB equips professionals to handle complex numerical data analysis and simulation tasks effectively.
6. **Stata**
- Stata is widely used in econometrics and biostatistics, providing a range of statistical functions and data management capabilities. Knowledge of Stata facilitates effective analysis and interpretation of data in these fields.
7. **Tableau**
- Tableau is a powerful data visualization tool that allows users to create interactive and shareable dashboards. Professionals should be adept at using Tableau to present data insights in a compelling manner to non-technical stakeholders.
8. **Excel for Statistical Analysis**
- Excel remains a foundational tool for data analysis, featuring various statistical functions and pivot tables. Mastering Excel enables professionals to perform basic analyses, manage datasets, and visualize results quickly.
9. **Power BI**
- Power BI is a business analytics tool that allows users to visualize data and share insights across their organization. Understanding Power BI enables professionals to create reports and dashboards that communicate data findings effectively.
10. **SQL (Structured Query Language)**
- SQL is critical for managing and retrieving data from relational databases. Professionals with SQL skills can write complex queries to extract, manipulate, and analyze data, forming the backbone of many data-driven projects.
11. **Hadoop and R**
- Familiarity with big data frameworks like Hadoop, combined with R, allows professionals to analyze large datasets efficiently. This skill is increasingly important in industries dealing with large volumes of structured and unstructured data.
12. **Apache Spark**
- Apache Spark is used for big data processing and analytics, providing an interface for programming entire clusters with implicit data parallelism and fault tolerance. Knowledge of Spark helps professionals analyze and process data at scale quickly.
13. **TensorFlow for Statistical Learning**
- TensorFlow is a deep learning framework that also offers powerful statistical learning capabilities. Professionals with TensorFlow skills can build, train, and deploy machine learning models.
14. **Data Mining Techniques**
- Understanding data mining techniques is crucial for extracting patterns and insights from large datasets. Professionals skilled in data mining can identify trends, correlations, and anomalies that inform business strategies.
15. **Simulation Software (e.g., AnyLogic, Simul8)**
- Proficiency in simulation software allows professionals to model real-world processes and assess the impact of different scenarios. This skill is vital in operations research, logistics, and supply chain management.
16. **Graphical Modeling Tools (e.g., GraphPad Prism)**
- Graphical modeling tools help visualize complex data relationships through graphs and models. Professionals should be adept at using these tools to communicate findings and support decision-making.
17. **Bayesian Analysis Software (e.g., JAGS, Stan)**
- Bayesian analysis offers a powerful approach to statistical modeling. Familiarity with software like JAGS and Stan enables professionals to conduct advanced analyses that incorporate prior knowledge into decision-making frameworks.
18. **Web Scraping Tools (e.g., Beautiful Soup, Scrapy)**
- Web scraping tools are essential for gathering data from websites for analysis. Knowledge of these tools allows professionals to extract, clean, and prepare web-based data to support various analytical projects.
19. **Visualization Libraries (e.g., Matplotlib, Seaborn)**
- Proficiency in visualization libraries enhances the ability to create informative data visualizations. Skills in tools like Matplotlib and Seaborn are valuable for representing complex datasets in an understandable way, aiding in data interpretation and storytelling.
Mastering these hard skills will not only enhance a professional's capabilities in data analysis but also make them invaluable assets in any data-driven organization.
1. **Statistical Software Proficiency**: Expertise in using statistical software tools such as R, SAS, or SPSS for data analysis and interpretation.
2. **Data Visualization**: Ability to create compelling visual representations of data using tools like Tableau, Power BI, or matplotlib to effectively communicate findings.
3. **Database Management**: Proficient in SQL for database querying, manipulation, and management to extract relevant datasets for analysis.
4. **Advanced Excel Skills**: Strong skills in Microsoft Excel, including functions, pivot tables, and data modeling to manipulate and analyze large datasets.
5. **Programming Skills**: Knowledge of programming languages such as Python or R for data analysis, automation, and building predictive models.
6. **Statistical Analysis Techniques**: Understanding and application of statistical methods, such as regression analysis, hypothesis testing, and A/B testing.
7. **Data Cleaning and Preprocessing**: Capable of cleaning and preparing data for analysis, ensuring accuracy and consistency in datasets by using techniques such as data wrangling and normalization.
Generate Your Cover letter Summary with AI
Accelerate your Cover letter crafting with the AI Cover letter Builder. Create personalized Cover letter summaries in seconds.
Related Resumes:
Generate Your NEXT Resume with AI
Accelerate your Resume crafting with the AI Resume Builder. Create personalized Resume summaries in seconds.